2 An Integrative Dual Systems Approach to Digital Self-Control
In the rest of the paper, we draw on dual systems theory and argue how it can be used to systematise and classify digital self-control tools. We start by outlining the basics of the underlying psychological research. In doing so, we extend current applications of dual system theories in DBCIs (Pinder et al. 2018, 2017; Cox et al. 2016; Adams et al. 2015) with the concept of ‘expected value of control’, which the neuroscience literature considers central in explaining why success at self-control fluctuates over time and with emotional state (Shenhav, Botvinick, and Cohen 2013; Botvinick and Braver 2015; Inzlicht, Schmeichel, and Macrae 2014; Ryan et al. 2014; Hendershot et al. 2011; Tice, Bratslavsky, and Baumeister 2001). An overview of the resulting model is shown in Figure 2.1.
Subsequently, we review current digital self-control tools on the Chrome Web, Google Play, and Apple App stores, and apply the model to organise and explain common design features, before pointing out gaps and opportunities for future work.
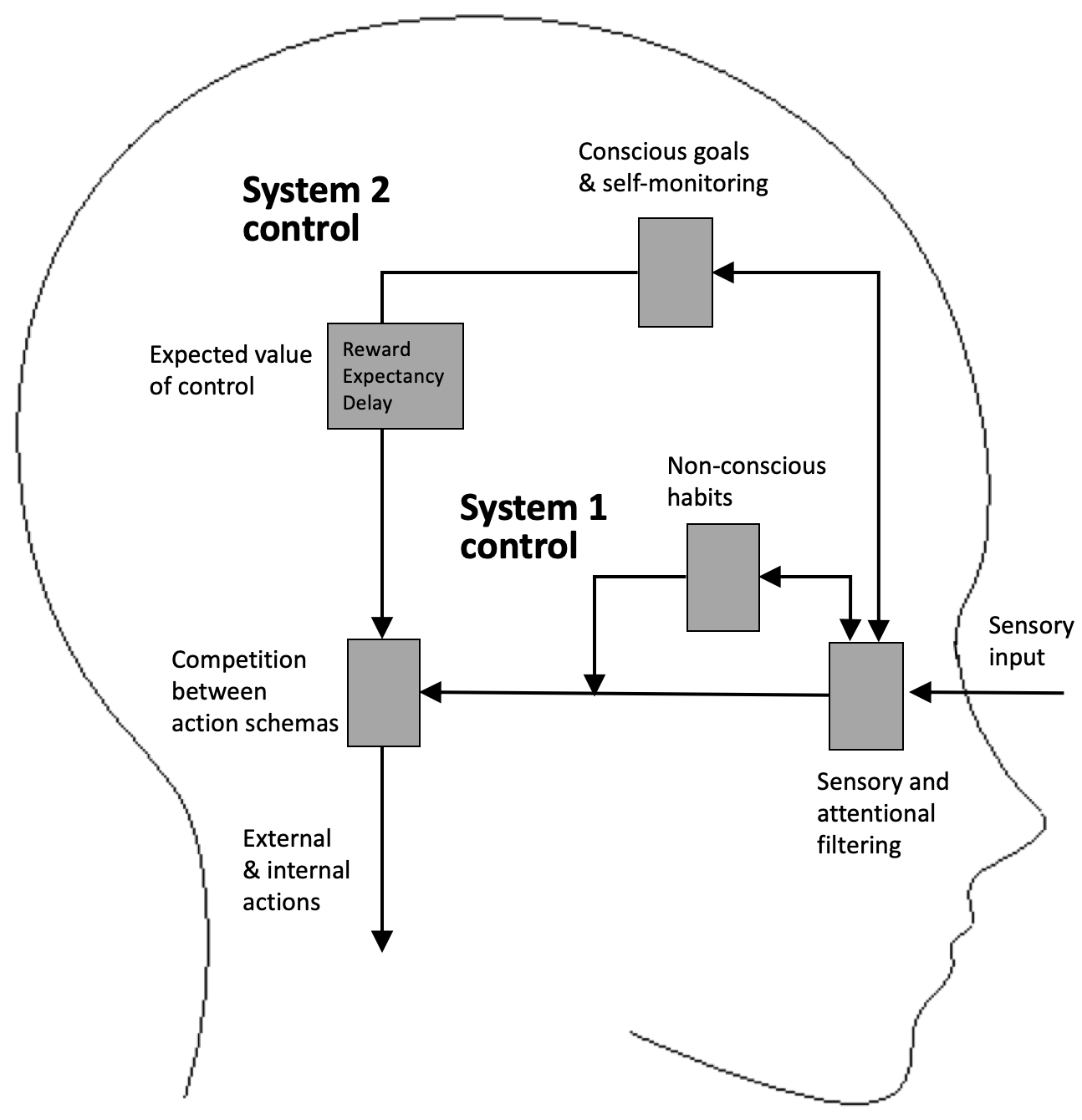
Figure 2.1: An extended dual systems model of self-regulation, developed from Shea et al. (Shea et al. 2014) and Norman & Shallice (Norman and Shallice 1986). System 1 control is rapid and non-conscious, whereas System 2 control is slower, conscious, and capacity-limited. The strength of System 2 control is mediated by the expected value of control.
2.1 System 1 and System 2
The core of dual systems theories is a major distinction between swift, parallel and non-conscious ‘System 1’ processes, and slower, capacity-limited and conscious ‘System 2’ processes (Kahneman 2011; Smith and DeCoster 2000; Shiffrin and Schneider 1977; Miller and Cohen 2001; Miller and D’Esposito 2005; Shea et al. 2014; Cooper, Ruh, and Mareschal 2014).2
According to this model, System 1 control is driven by environmental inputs and internal states along with cognitive pathways that map the current situation to well-learned habits or instinctive responses (Miller and Cohen 2001). Behaviour driven by System 1 is often called ‘automatic’, as it allows tasks to be initiated or performed without conscious awareness and with little interference with other tasks (Norman and Shallice 1986). Instinctive responses like scratching mosquito bites, or frequent patterns of digital device use like picking up one’s smartphone to check for notifications, can happen automatically via System 1 control (cf. Van Deursen et al. 2015; Oulasvirta et al. 2012; Botvinick and Braver 2015).
System 2 control is driven by goals, intentions, and rules held in conscious working memory (Miller and D’Esposito 2005; Baddeley 2003). From these central representations, signals are sent to cognitive systems that process sensory input, memory retrieval, emotional processing, and behavioural output, to guide responses accordingly (Miller and Cohen 2001). System 2 control is necessary when a goal requires planning or decision-making, or overcoming of habitual responses or temptations (Norman and Shallice 1986), for example if one has a goal of not scratching mosquito bites or not checking a smartphone notification.
2.2 Action schema competition
From a neuroscience perspective, the building blocks of behaviour are hierarchical action schemas, that is, control units for partially ordered sequences of action that achieve some goal when performed in the appropriate order (Norman and Shallice 1986; Cooper and Shallice 2000). Action schemas exist at varying levels of complexity, from simple single-action motor schemas for grasping and twisting, to higher level schemas for e.g. preparing tea by filling and boiling a kettle (Shallice and Cooper 2011; Botvinick 2008). The schemas compete for control over behaviour in a ‘competitive selection’ process in which schemas act like nodes in a network, each with a continuous activation value (Shallice and Cooper 2011), and the ‘winner’ is the node with the strongest activation (Knudsen 2007; Pinder et al. 2018). Schema nodes are activated by a number of sources, including sensory input via System 1 processes (‘bottom-up’), ‘parental’ influence from super-ordinate schemas in the hierarchy, and top-down influence from System 2 control (Cooper and Shallice 2000; Shallice and Cooper 2011).
2.3 Self-regulation and self-control
Following others, we use self-regulation as an umbrella term for regulatory processes in the service of goal-directed behaviour, including automatic System 1 habits, and self-control more restrictively for conscious and deliberate System 2 control in situations where immediate impulses conflict with enduringly valued goals (Hagger et al. 2010; Baumeister, Vohs, and Tice 2007; Milyavskaya and Inzlicht 2018; Duckworth, Gendler, and Gross 2014). For example, if a person wishes to be less distracted by her smartphone in social situations, and through repetition has acquired a habit of turning the phone face-down to the point that she now does it without conscious attention, this counts as self-regulation. If in a given moment she feels an urge to flip it over and check for notifications, but consciously suppresses this impulse and does not act on it, this counts as self-control.
Self-regulation and self-control are mediated by feedback functions for monitoring the state of oneself and the environment, comparing this state to goals and standards (cf. Shea et al. 2014), and acting to modify the situation accordingly (Carver and Scheier 1998, cf. cybernetic models of behaviour control, 1981; Baumeister and Exline 2000; Inzlicht, Legault, and Teper 2014; Powers 1973).
2.4 Attentional filtering
For goals, rules, or intentions to guide System 2 control, they must first enter working memory (Baddeley 2003; Miller and D’Esposito 2005). Entry of information from the external world, internal states, or memory stores into working memory is itself a competitive process, in which the signals with the highest activation values are given access by attentional filters (Constantinidis and Wang 2007; Miller and Cohen 2001; Knudsen 2007).
Automatic bottom-up filters look out for stimulus properties that are likely to be important, either through innate sensitivities (e.g. sudden or looming noises) or learned associations (e.g. a smartphone notification) and boost their signal strength (Knudsen 2007). In this way, some stimuli may evoke a response strong enough to gain automatic access to working memory even while we have our minds on other things (Itti and Koch 2001; Egeth and Yantis 1997). For example, clickbait uses headlines and imagery with properties that makes bottom-up attention filters put its information on a fast track to conscious working memory or trigger click-throughs via System 1 control (cf. Blom and Hansen 2015).
Conscious System 2 control can also direct attention towards particular internal or external sources of information (e.g. focusing on a distorted voice in one’s cellphone on a crowded train), which increases the signal strength of those sources and makes the information they carry more likely to enter working memory (Corbetta et al. 1991; Müller, Philiastides, and Newsome 2005; Knudsen 2007; Shu, Hasenstaub, and McCormick 2003).
2.5 Self-control limitations and the Expected Value of Control
A central puzzle is why people often fail to act in accordance with their own valued goals, even when they are aware of the mismatch (Duckworth, Gendler, and Gross 2016). According to current research on cognitive control, the two key factors to answer this question are (i) limitations on System 2 control in relation to capacity; and (ii) fluctuations due to emotional state and fatigue (Botvinick and Braver 2015).
Capacity limitations
The amount of information that can be held in working memory and guide System 2 control, is limited (classically ‘seven, plus or minus two’ chunks of meaningful information (Miller 1956; Cowan 2010)). Therefore, self-control can fail if the relevant goals are simply not represented in working memory at the time of action (Kotabe and Hofmann 2015). This is one explanation for why people often struggle to manage use of e.g. Facebook or email - one opens the application with a particular goal in mind, but information from the news feed or inbox hijacks attention and crowds out the initial goal.
Fluctuations due to emotional state and fatigue
System 2 control often suffers from fatigue effects if exerted continuously (Blain, Hollard, and Pessiglione 2016; Dai et al. 2015; Hockey 2013; Hagger et al. 2010) and also fluctuates with emotional state (Tice, Bratslavsky, and Baumeister 2001). For example, negative mood is a strong predictor of relapse of behaviour people attempt to avoid (Miller et al. 1996; Hendershot et al. 2011; Tice, Bratslavsky, and Baumeister 2001), and studies of Facebook use have found that users are worse at regulating the time they spend on the platform when in a bad mood (Ryan et al. 2014; cf. Montag et al. 2017).
The emerging consensus explanation of these fluctuations is that the strength of System 2 control is mediated by a cost-benefit analysis of the outcome that exercising self-control might bring about (Botvinick and Braver 2015) i.e., the expected value of control (EVC) (Shenhav, Botvinick, and Cohen 2013)3. The research suggests that EVC is influenced by at least three major factors:
First, EVC increases the more reward people perceive they could obtain (or the greater the loss that could be avoided) through successful self-control (Botvinick and Braver 2015; Padmala and Pessoa 2010, 2011; Adcock et al. 2006). To illustrate, consider ‘phone stack’, in which a group dine at a restaurant and begin by stacking up their phones on the table. The first person to take out his phone from the stack to check it, has to pay the entire bill (Ha 2012; Tell 2013). This game aids self-control over device use by introducing a financial (and reputational) cost which adjusts the overall expected value of control (cf. also Ko et al.’s tool NUGU (Ko et al. 2015)).
Second, EVC increases the greater expectancy, or perceived likelihood, that one will be able to bring a given outcome about through self-control (Vroom 1964; Bernoulli 1954; Steel and König 2006; cf. “self-efficacy” in Social Cognitive Theory, Bandura 1991). In the phone stack example, people may try harder to suppress an urge to check their phone, the more confidence they have in their ability to control themselves in the first place.
Third, EVC decreases the longer the delay before the outcome that self-control might bring about (cf. ‘future discounting’ (Critchfield and Kollins 2001; Ainslie 2001, 2010; Evans et al. 2016; Ariely and Wertenbroch 2002; McClure et al. 2007; O’Donoghue and Rabin 2001)). In phone stack, we should expect people to be worse at suppressing an impulse to check their phone if the rules were changed so that the loser would pay the bill for a meal in 10 years’ time.
2.6 A practical example
As an illustration of the model and the benefits of including EVC, consider a student who opens his laptop to work on an essay. However, he instead checks Facebook and spends an inordinate amount of time scrolling the news feed, experiencing feelings of regret having done so when he finally returns to the essay. This is not the first time it happened, even though his reflective goal is always to do solid work on the essay as the first thing, and to only allow himself to check Facebook briefly during breaks.
Our model suggests that we think about this situation in terms of the perceptual cues in the context, automatic System 1 behaviour control, System 2’s consciously held goals and self-monitoring, and System 2’s expected value of control:
If the student normally checks Facebook when opening his laptop, this context may trigger a habitual check-in via System 1 control. His goal of working first thing might be present in his working memory, but he might fail to override his checking habit due to his expected value of control being low. This might be because he did not get any reward from inhibiting the impulse to check Facebook; because he had little confidence in his own ability to suppress this urge (low expectancy); or because the rewards from working on his essay were delayed because it was only due in two months. Alternatively, his goal of working on the essay first thing might not be present in his working memory, in which case no System 2 control was initiated to override the checking habit.
After having opened Facebook, he might remember that he should be working on the essay, but attention-grabbing content from the newsfeed enters his capacity-limited working memory and crowd out this goal, leading him to spend more time on Facebook than intended.
References
Pinder, Charlie, Jo Vermeulen, Benjamin R. Cowan, and Russell Beale. 2018. “Digital Behaviour Change Interventions to Break and Form Habits.” ACM Transactions on Computer-Human Interaction 25 (3): 1–66. https://doi.org/10.1145/3196830.
Pinder, Charlie, Jo Vermeulen, Benjamin R. Cowan, Russell Beale, and Robert J. Hendley. 2017. “Exploring the feasibility of subliminal priming on smartphones.” In Proceedings of the 19th International Conference on Human-Computer Interaction with Mobile Devices and Services - Mobilehci ’17. https://doi.org/10.1145/3098279.3098531.
Cox, Anna L, Sandy J J Gould, Marta E Cecchinato, Ioanna Iacovides, and Ian Renfree. 2016. “Design Frictions for Mindful Interactions: The Case for Microboundaries.” In Proceedings of the 2016 Chi Conference Extended Abstracts on Human Factors in Computing Systems, 1389–97. CHI Ea ’16. New York, NY, USA: ACM. https://doi.org/10.1145/2851581.2892410.
Adams, Alexander T., Jean Costa, Malte F. Jung, and Tanzeem Choudhury. 2015. “Mindless Computing: Designing Technologies to Subtly Influence Behavior.” In Proceedings of the 2015 Acm International Joint Conference on Pervasive and Ubiquitous Computing, 719–30. ACM. https://doi.org/10.1145/2750858.2805843.
Shenhav, Amitai, Matthew M Botvinick, and Jonathan D Cohen. 2013. “The Expected Value of Control: An Integrative Theory of Anterior Cingulate Cortex Function.” Neuron 79 (2): 217–40. https://doi.org/https://doi.org/10.1016/j.neuron.2013.07.007.
Botvinick, Matthew, and Todd S Braver. 2015. “Motivation and cognitive control: From behavior to neural mechanism.” Annual Review of Psychology 66: 83–113. https://doi.org/10.1146/annurev-psych-010814-015044.
Inzlicht, Michael, Brandon J. Schmeichel, and C. Neil Macrae. 2014. “Why self-control seems (but may not be) limited.” Trends in Cognitive Sciences 18 (3). Elsevier Ltd: 127–33. https://doi.org/10.1016/j.tics.2013.12.009.
Ryan, Tracii, Andrea Chester, John Reece, and Sophia Xenos. 2014. “The uses and abuses of Facebook: A review of Facebook addiction.” Journal of Behavioural Addictions 3 (3): 133–48. https://doi.org/10.1556/JBA.3.2014.016.
Hendershot, Christian S, Katie Witkiewitz, William H George, and G Alan Marlatt. 2011. “Relapse prevention for addictive behaviors.” Substance Abuse Treatment, Prevention, and Policy 6 (1): 17. https://doi.org/10.1186/1747-597X-6-17.
Tice, D M, E Bratslavsky, and R F Baumeister. 2001. “Emotional distress regulation takes precedence over impulse control: if you feel bad, do it!” Journal of Personality and Social Psychology 80 (1): 53–67. https://doi.org/10.1037/0022-3514.80.1.53.
Shea, Nicholas, Annika Boldt, Dan Bang, Nick Yeung, Cecilia Heyes, and Chris D Frith. 2014. “Supra-personal cognitive control and metacognition.” Trends in Cognitive Sciences 18 (4). Elsevier Ltd: 186–93. https://doi.org/10.1016/j.tics.2014.01.006.
Norman, D.A., and T. Shallice. 1986. “Attention to Action: Willed and Automatic Control of Behaviour.” In Consciousness and Self-Regulation (Advances in Research and Theory, Vol. 4), edited by R.J. et al. Davidson, 1–18. Plenum.
Kahneman, Daniel. 2011. Thinking, fast and slow. New York: Allen Lane (Penguin).
Smith, Eliot R., and Jamie DeCoster. 2000. “Dual-Process Models in Social and Cognitive Psychology: Conceptual Integration and Links to Underlying Memory Systems.” Personality and Social Psychology Review 4 (2): 108–31. https://doi.org/10.1207/S15327957PSPR0402_01.
Shiffrin, Richard M., and Walter Schneider. 1977. “Controlled and automatic human information processing: II. Perceptual learning, automatic attending and a general theory.” Psychological Review 84 (2): 127–90. https://doi.org/10.1037/0033-295X.84.2.127.
Miller, E K, and J D Cohen. 2001. “An integrative theory of prefrontal cortex function.” Annual Review of Neuroscience 24 (1): 167–202. https://doi.org/10.1146/annurev.neuro.24.1.167.
Miller, Brian T., and Mark D’Esposito. 2005. “Searching for "the top" in top-down control.” Neuron 48 (4): 535–38. https://doi.org/10.1016/j.neuron.2005.11.002.
Cooper, Richard P., Nicolas Ruh, and Denis Mareschal. 2014. “The goal circuit model: A hierarchical multi-route model of the acquisition and control of routine sequential action in humans.” Cognitive Science 38 (2): 244–74. https://doi.org/10.1111/cogs.12067.
Van Deursen, Alexander J.A.M., Colin L. Bolle, Sabrina M. Hegner, and Piet A.M. Kommers. 2015. “Modeling habitual and addictive smartphone behavior: The role of smartphone usage types, emotional intelligence, social stress, self-regulation, age, and gender.” Computers in Human Behavior 45 (April). Elsevier Ltd: 411–20. https://doi.org/10.1016/j.chb.2014.12.039.
Oulasvirta, Antti, Tye Rattenbury, Lingyi Ma, and Eeva Raita. 2012. “Habits make smartphone use more pervasive.” Personal and Ubiquitous Computing 16 (1): 105–14. https://doi.org/10.1007/s00779-011-0412-2.
Baddeley, Alan. 2003. “Working Memory: Looking Back and Looking Forward.” Nature Reviews. Neuroscience 4 (October): 829–39.
Cooper, Richard, and Tim Shallice. 2000. “Contention Scheduling and the Control of Routine Activities.” Cognitive Neuropsychology 17 (4): 297–338. https://doi.org/10.1080/026432900380427.
Shallice, Tim, and Richard P. Cooper. 2011. The Organisation of Mind. Oxford: Oxford University Press.
Botvinick, Matthew M. 2008. “Hierarchical models of behaviors and prefrontal function.” Trends in Cognitive Sciences 12 (5): 201–8. https://doi.org/10.1016/j.tics.2008.02.009.
Knudsen, Eric I. 2007. “Fundamental Components of Attention.” Annual Review of Neuroscience 30: 57–78. https://doi.org/10.1146/annurev.neuro.30.051606.094256.
Hagger, Martin S, Chantelle Wood, Chris Stiff, and Nikos L D Chatzisarantis. 2010. “Ego depletion and the strength model of self-control: a meta-analysis.” Psychological Bulletin 136 (4): 495–525. https://doi.org/10.1037/a0019486.
Baumeister, Roy F., Kathleen D Vohs, and Dianne M Tice. 2007. “The Strength Model of Self-Control.” Current Directions in Psychological Science 16 (6): 351–55. https://doi.org/10.1111/j.1467-8721.2007.00534.x.
Milyavskaya, Marina, and Michael Inzlicht. 2018. “Attentional and Motivational Mechanisms of Self-Control.” Handbook of Self-Control in Health & Well- Being, 11–23. https://doi.org/10.1017/CBO9781107415324.004.
Duckworth, Angela L., Tamar Szabó Gendler, and James J. Gross. 2014. “Self Control in School Age Children.” Educational Psychologist 49 (3): 199–217. https://doi.org/10.1080/00461520.2014.926225.
Carver, C. 1998. On the self-regulation of behavior. New York: Cambridge University Press.
Carver, C. S., and M. F. Scheier. 1981. Attention and self-regulation: A control-theory approach to human behavior. New York: Springer-Verlag.
Baumeister, Roy F., and Julie Juola Exline. 2000. “Self-control, Morality, and Human Strength.” Journal of Social and Clinical Psychology 19 (1): 29–42.
Inzlicht, Michael, Lisa Legault, and Rimma Teper. 2014. “Exploring the Mechanisms of Self-Control Improvement.” Current Directions in Psychological Science 23 (4): 302–7. https://doi.org/10.1177/0963721414534256.
Powers, W. T. 1973. Behavior: The control of perception. Chicago: Aldine.
Constantinidis, Christos, and Xiao-Jing Wang. 2007. “A Neural Circuit Basis for Spatial Working Memory.” The Neuroscientist 10 (6): 553–65. https://doi.org/10.1177/1073858404268742.
Itti, L, and C Koch. 2001. “Computational modelling of visual attention.” Nature Reviews. Neuroscience 2 (3): 194–203. https://doi.org/10.1038/35058500.
Egeth, H E, and S Yantis. 1997. “Visual attention: control, representation, and time course.” Annual Review of Psychology 48: 269–97. https://doi.org/10.1146/annurev.psych.48.1.269.
Blom, Jonas Nygaard, and Kenneth Reinecke Hansen. 2015. “Click bait: Forward-reference as lure in online news headlines.” Journal of Pragmatics 76. Elsevier B.V.: 87–100. https://doi.org/10.1016/j.pragma.2014.11.010.
Corbetta, M, F M Miezin, S Dobmeyer, G L Shulman, and S E Petersen. 1991. “Selective and divided attention during visual discriminations of shape, color, and speed: functional anatomy by positron emission tomography.” The Journal of Neuroscience : The Official Journal of the Society for Neuroscience 11 (8): 2383–2402. http://www.ncbi.nlm.nih.gov/pubmed/1869921.
Müller, James R, Marios G Philiastides, and William T Newsome. 2005. “Microstimulation of the superior colliculus focuses attention without moving the eyes.” Proceedings of the National Academy of Sciences of the United States of America 102 (3): 524–9. https://doi.org/10.1073/pnas.0408311101.
Shu, Yousheng, Andrea Hasenstaub, and David A. McCormick. 2003. “Turning on and off recurrent balanced cortical activity.” Nature 423 (May): 288–93. https://doi.org/10.1038/nature01614.1.
Duckworth, A. L., T. S. Gendler, and J. J. Gross. 2016. “Situational Strategies for Self-Control.” Perspectives on Psychological Science 11 (1). https://doi.org/10.1177/1745691615623247.
Miller, George A. 1956. “The magical number seven, plus or minus two: some limits on our capacity for processing information.” Psychological Review 63 (2): 343–52. https://doi.org/10.1037/h0043158.
Cowan, Nelson. 2010. “The Magical Mystery Four: How is Working Memory Capacity Limited, and Why?” Curr Dir Psychol Sci 19 (1): 51–57. https://doi.org/10.1177/0963721409359277.
Kotabe, Hiroki P., and Wilhelm Hofmann. 2015. “On Integrating the Components of Self-Control.” Perspectives on Psychological Science 10 (5): 618–38. https://doi.org/10.1177/1745691615593382.
Blain, Bastien, Guillaume Hollard, and Mathias Pessiglione. 2016. “Neural mechanisms underlying the impact of daylong cognitive work on economic decisions.” Proceedings of the National Academy of Sciences 113 (25): 6967–72. https://doi.org/10.1073/pnas.1520527113.
Dai, Hengchen, Katherine L. Milkman, David A. Hofmann, and Bradley R. Staats. 2015. “The impact of time at work and time off from work on rule compliance: The case of hand hygiene in health care.” Journal of Applied Psychology 100 (3): 846–62. https://doi.org/10.1037/a0038067.
Hockey, Robert. 2013. The Psychology of Fatigue: Work, Effort and Control. Cambridge University Press. https://doi.org/10.1017/CBO9781139015394.
Miller, W. R., V. S. Westerberg, R. J. Harris, and J. S. Tonigan. 1996. “What predicts relapse? Prospective testing of antecedent models.” Addiction 91 (SUPPL.). https://doi.org/10.1111/j.1360-0443.1996.tb02336.x.
Montag, Christian, Alexander Markowetz, Konrad Blaszkiewicz, Ionut Andone, Bernd Lachmann, Rayna Sariyska, Boris Trendafilov, et al. 2017. “Facebook usage on smartphones and gray matter volume of the nucleus accumbens.” Behavioural Brain Research 329 (April): 221–28. https://doi.org/10.1016/j.bbr.2017.04.035.
Padmala, Srikanth, and Luiz Pessoa. 2010. “Interactions between cognition and motivation during response inhibition.” Neuropsychologia 48 (2): 558–65. https://doi.org/10.1016/j.neuropsychologia.2009.10.017.
Padmala, Srikanth, and Luiz Pessoa. 2011. “Reward reduces conflict by enhancing attentional control and biasing visual cortical processing.” Journal of Cognitive Neuroscience 23 (11): 3419–32. https://doi.org/10.1162/jocn_a_00011.
Adcock, R. Alison, Arul Thangavel, Susan Whitfield-Gabrieli, Brian Knutson, and John D.E. Gabrieli. 2006. “Reward-Motivated Learning: Mesolimbic Activation Precedes Memory Formation.” Neuron 50 (3): 507–17. https://doi.org/10.1016/j.neuron.2006.03.036.
Ha, Anthony. 2012. “The Phone Stacking Game: Let’s Make This A Thing.” https://techcrunch.com/2012/02/04/the-phone-stacking-game-lets-make-this-a-thing/.
Tell, Caroline. 2013. “Step Away From the Phone!” https://www.nytimes.com/2013/09/22/fashion/step-away-from-the-phone.html.
Ko, Minsam, Kyong-Mee Chung, Subin Yang, Joonwon Lee, Christian Heizmann, Jinyoung Jeong, Uichin Lee, Daehee Shin, Koji Yatani, and Junehwa Song. 2015. “NUGU: A Group-based Intervention App for Improving Self-Regulation of Limiting Smartphone Use.” Proceedings of the 18th ACM Conference on Computer Supported Cooperative Work & Social Computing - CSCW ’15, 1235–45. https://doi.org/10.1145/2675133.2675244.
Vroom, V. H. 1964. Work and Motivation. New York: Wiley.
Bernoulli, D. 1954. “Exposition of a new theory of the measurement of risk.” Econometrica 22: 23–36.
Steel, Piers, and Cornelius J. König. 2006. “Integrating theories of motivation.” Academy of Management Review 31 (4): 889–913. https://doi.org/10.5465/AMR.2006.22527462.
Bandura, Albert. 1991. “Social Cognitive Theory of Self-Regulation.” Organizational Behavior and Human Decision Processes 50 (2): 248–87. https://doi.org/10.1016/0749-5978(91)90022-L.
Critchfield, T S, and S H Kollins. 2001. “Temporal discounting: basic research and the analysis of socially important behavior.” Journal of Applied Behavior Analysis 34 (1): 101–22. https://doi.org/10.1901/jaba.2001.34-101.
Ainslie, G. 2001. Breakdown of Will. Cambridge University Press.
Ainslie, G. 2010. “Procrastination: The Basic Impulse.” In The Thief of Time: Philosophical Essays on Procrastination, edited by Andreou Chrisoula and Mark D. White, 11–27. New York: Oxford University Press.
Evans, Owain, Andreas Stuhl, Noah D. Goodman, Andreas Stuhlmüller, and Noah D. Goodman. 2016. “Learning the Preferences of Ignorant, Inconsistent Agents.” Proceedings of the Thirtieth AAAI Conference on Artificial Intelligence, 323–29.
Ariely, Dan, and Klaus Wertenbroch. 2002. “Procrastination, deadlines, and performance: self-control by precommitment.” Psychological Science, no. 2000: 219–24. https://doi.org/10.1111/1467-9280.00441.
McClure, S. M., K. M. Ericson, D. I. Laibson, G. Loewenstein, and J. D. Cohen. 2007. “Time Discounting for Primary Rewards.” Journal of Neuroscience 27 (21): 5796–5804. https://doi.org/10.1523/JNEUROSCI.4246-06.2007.
O’Donoghue, Ted, and Matthew Rabin. 2001. “Choice and Procrastination.” Quarterly Journal of Economics 116 (1): 121–60. https://doi.org/10.1162/003355301556365.
The related ‘Nudge theory’ (Sunstein and Thaler 2008) draws upon dual systems theories to describe how to exploit System 1 and sometimes System 2 processes to guide people towards a desired action (cf. Adams et al. 2015).↩
The alternative and influential ‘ego-depletion’ explanation (Baumeister, Vohs, and Tice 2007) suggests that System 2 control relies on a limited resource that could be depleted, and which has a ‘refraction period’ before optimal self-control can again be exercised (Hagger et al. 2010). This model has intuitive appeal but has not withstood scrutiny (Lurquin and Miyake 2017), as original studies have failed to replicate (Xu et al. 2014; Hagger et al. 2016), depletion effects have shown to be reversible by increasing the rewards for sustained performance (Muraven and Slessareva 2003; Hagger et al. 2010), and the purported physical resource underlying the effect failed to be discovered (Lange and Eggert 2014; Molden et al. 2012).↩