2 Background and Motivation
In this chapter, we summarise the background and motivation of the present thesis:
First, we review evidence on the existence of self-control struggles in relation to digital device use.
Next, we consider what criteria of ‘success’ design patterns might be benchmarked against, summarising HCI research attempting to bridge the gap between what users actually do and what they ‘really wanted’, as well as main insights from basic research on the nature of self-control.
Afterwards, we zoom in on the existing studies of design patterns for digital self-control, reviewing their approaches, findings, and guiding theory.
Laying the ground for the subsequent chapters, we end by highlighting limitations of existing studies and pointing to the thesis chapters that explore corresponding research opportunities.
2.1 Digital device use and self-control challenges
2.1.1 Behavioural addiction
It has long been posited that use of Information Communication Technologies (ICTs) for some subset of users can become associated with severe breakdowns of self-regulation, causing distress or impaired functioning in important life domains (Chakraborty, Basu, and Vijaya Kumar 2010). For more than two decades, the concept of ‘addiction’ has been applied by some researchers to such instances, originally in the form of ‘internet addiction’ (K. S. Young 1998), and more recently ‘cell phone’ and ‘smartphone addiction’ (Chakraborty, Basu, and Vijaya Kumar 2010; Sapacz, Rockman, and Clark 2016), as well as ‘social media’ or ‘Facebook addiction’ (Andreassen et al. 2016; Marino et al. 2018b, 2018a; Ryan et al. 2014; Sleeper et al. 2015). This research tends to focus on a more restricted subset of self-control difficulties than the present thesis, namely struggles with controlling digital device use that are so severe that they result in real negative life impact, where a clinical term may be appropriate. However, because the concept of ‘addiction’ has often been applied somewhat loosely — and because narratives of addiction have had a significant influence on public discourse and users’ personal reflections (Lanette and Mazmanian 2018; Tiku 2018; Orben 2019) — this research space provides a useful point of departure.
‘Internet addiction’ has been defined as ‘time-consuming computer usage that causes distress or impairs functioning in important life domains’ (Chakraborty, Basu, and Vijaya Kumar 2010), mobile phone addiction as ‘repetitive use of mobile phones to engage in behaviour known to be counterproductive to health’ (Lopez-Fernandez et al. 2014), and ‘Facebook addiction’ (also ‘Facebook dependence’ or simply ‘Problematic Facebook Use’) as ‘problematic behaviour characterised by addictive-like symptoms and/or self-regulation difficulties related to Facebook use leading to negative consequences in personal and social life’ (Marino et al. 2018a). Early work on internet addiction found that ‘addicts’ on average spent 8 times as much time online as ‘non-addicts’ and reported problems akin to substance abuse, including negative consequences on educational, work, and personal life, inability to break problematic usage patterns, and withdrawal symptoms when unable to access the internet. `Addicts’ also tended to be heavy users of online chat rooms and multiplayer role playing games, suggesting that addictive use was driven by interactivity and need-provision online rather than “The Internet” as such (cf. more recent work describing social networking sites as “addiction prone technologies,” Tarafdar, Gupta, and Turel (2013); Turel and Serenko (2012); Ryan et al. (2014)). Similarly, research on cell phone addiction has found that users classified as ‘addicts’ experience withdrawal symptoms in absence of their device — feelings of loss, signs of craving, and functional impairment — and a resulting loss of control in managing other activities (Cheever et al. 2014; Lopez-Fernandez et al. 2014; Roberts, Pullig, and Manolis 2015).
However, applying the concept of addiction to ICT use has been enveloped in controversy. Initial work on ‘internet addiction’ modified the American Psychiatric Association’s DSM-IV diagnostic criteria for pathological gambling (K. S. Young 1998), and came to describe it in terms of four components: i) excessive use (often associated with a loss of sense of time or neglect of basic drives), ii) withdrawal symptoms when one’s computer is inaccessible (e.g. anger, tension, or depression), iii) tolerance (e.g. increased hours of use and increased expense on equipment over time), and iv) negative repercussions (e.g. social isolation, fatigue, lying to one’s surroundings about use, poor achievement, etc.) (Tao et al. 2010; Beard and Wolf 2001; Chakraborty, Basu, and Vijaya Kumar 2010). However, the subsequent literature has seen little agreement on how to delineate the boundaries of addictive use, and researchers have often used terms such as ‘addiction,’ ‘abuse,’ and ‘problematic use’ interchangeably (Gutiérrez, Rodriguez de Fonseca, and Rubio 2016). As a result, a wide range of prevalence estimates for addictive use has been given: ‘internet addiction’ has been suggested to affect anywhere between 0.3 and 38% of populations in developed economies (Chakraborty, Basu, and Vijaya Kumar 2010; C. Cheng and Li 2014; Weinstein et al. 2014), ‘smartphone addiction’ similarly between 0 and 38% (Lopez-Fernandez et al. 2014; Pedrero, Rodríguez, and Ruiz 2012), and ‘Problematic Facebook Use’ from 3.1% to 47% (J. Cheng, Burke, and Davis 2019; Jafarkarimi et al. 2016; see also Bányai et al. 2017; Khumsri et al. 2015; Wolniczak et al. 2013). Accordingly, some researchers have pushed back against a perceived pathologisation of everyday patterns of ICT use in this literature, and argued that even frequent, extensive use which distract users from daily life should not be considered “addiction” unless it also leads to functional impairment and psychological distress (Billieux et al. 2015; Kardefelt-Winther et al. 2017; Tran et al. 2019).
For the purposes of the present thesis, this literature suggests severe self-control difficulties in relation to digital device use among some smaller subset of users. Moreover, the high prevalence estimates of ‘addictive’ use in studies that apply less strict inclusion criteria suggests that milder struggles with self-control are widespread.
2.1.2 Common self-control struggles
The current surge of public discussion around self-control struggles and unwanted distraction — and the recent related initiatives by some of the tech giants (Apple 2018; Gonzalez 2018; Google 2018) — have a broader focus, namely daily self-control struggles assumed to be experienced by many, if not most, users (cf. Centers 2018; Wolwerton 2018). Thus, a wealth of articles and opinion pieces have in recent years appeared on the topic in major news outlets, viral blog posts, and popular science books (e.g., Alter 2017; Eyal 2019; Foer 2016; Harris 2016; Knapp 2013; Popescu 2018; Wu 2016). Empirical research suggests that this does not merely reflect another ‘moral panic’ over new technology in society (cf. Lanette and Mazmanian 2018; Orben 2019): whereas it is unclear how often habits of digital device use meet clinical definitions of addiction, there is wide support for the claim that a high proportion of people regularly experience difficulties with self-control (Hiniker et al. 2016; Ko et al. 2016, 2015; Lundquist, Lefebvre, and Garramone 2014; Tran et al. 2019; Whittaker et al. 2016).
Initial work on intentional ‘non-use’ looked into why some users quit, or take breaks from Facebook (Baumer and Adams 2013), Twitter (Schoenebeck 2014), or other social networking sites. This work found that a major motivation for disengaging was struggles with distraction and self-control, with, e.g., users reporting deactivating Facebook because they found it ‘too interesting,’ or ‘superficial yet addictive’ and felt a need to delete or deactivate their accounts to concentrate on work or break out of a perceived addiction (Baumer and Adams 2013).
Most recent research has focused on smartphone use, where a number of studies have found broad frustration among ordinary users with their usage habits, particularly in relation to self-control struggles with high-reward, low-demand experiences such as games and browsing social media (Lukoff et al. 2018; Tran et al. 2019; Ames 2013; U. Lee et al. 2014; Shin and Dey 2013). For example, in a survey by Ko et al. (2015) posted in large online communities, a majority of smartphone users felt they were overusing their devices (64%) and wanted to change their usage habits (60%). The patterns respondents wished to change clustered around two themes: too frequent short usage, where incoming notifications or urges to, e.g., check the news derailed focus from tasks they wished to complete; and excessive long usage, where, e.g., habitually checking devices before bedtime ‘sucked them in’ (see also Ko et al. 2016; Oulasvirta et al. 2012). Most users also reported that their strategies for changing this behaviour most often failed, especially when relying on ‘willpower,’ because good intentions to limit use tended to be overridden by momentary impulses (Ko et al. 2015; cf. Elhai et al. 2016; Hofmann, Vohs, and Baumeister 2012; Lim et al. 2017).
In a survey of American smartphone users recruited on MTurk, 58% said they wanted to spend less time using their phones (Hiniker et al. 2016). Elaborating on their responses, many said they wanted to make context-dependent changes to their use, such as use their phones less before bedtime, or restructure time spent so that, e.g., their proportion of time in work-related activities were higher during commutes (Hiniker et al. 2016; see also Przybylski and Weinstein 2017).
In a recent study by Tran et al. (2019), which interviewed American participants from three subpopulations (high school students, college students, and post-graduation adults), participants reported filling every moment of downtime with habitual smartphone checking, which they engaged in with minimal awareness. They expressed frustration with their checking habits, particularly in relation to apps that drove them into compulsive use without adding enduring value to their lives, and most had tried regulating their use by deleting such apps after a sense of frustration built up.
Finally, related work on multitasking and media use has found that people often perceive their use of internet services to be in conflict with other important goals (Rosen, Mark Carrier, and Cheever 2013; S. Xu, Wang, and David 2016; Reinecke et al. 2018). For example, an experience sampling study of adults in the US questioned participants at random times during the day about whether and how they were currently using media (Reinecke and Hofmann 2016). In 51% of sampled episodes, participants were engaged in media use, with internet use the most common form (55% of instances; the most frequent content was social media, including Facebook and Twitter, video streaming sites, including YouTube, and online news sites). Importantly, participants reported that their use conflicted with other important goals on more than half of all use occurrences (61%) suggesting a high prevalence of self-control struggles with digital media use.
Some recent work on associations between device use and measures of well-being has called for careful examination of when self-reported frustration over, e.g., habitual smartphone use reflect users’ own lived experience, and when it reflects internalised narratives of, e.g., distracted addicts neglecting a morally ‘superior’ external world (Harmon and Mazmanian 2013; Orben 2019). This is an important point, and the study mentioned above by Tran et al. (2019) was specifically conducted as such a deeper examination among a random sample of smartphone users. Tran et al. (2019) found that, in addition to reporting general frustration with aspects of smartphone use they experienced as compulsive, participants also critically reflected on what use was meaningful and what was not, and described steps they had taken to minimise specific usage that did not provide them value. Whereas the influence on participant self-report of larger social narratives in relation to addictive use should be kept in mind, this research evidence suggests that reports of self-control struggles do reflect real aspects of users’ lived experience.
2.1.3 Challenges from constant connectivity and the attention economy
It is to be expected that tools which provide instant and permanent access to endless information, entertainment, and social connection come with self-control challenges. Thus, psychologists studying self-control have long known that relying on conscious willpower as self-control strategy in environments where distractions and temptations are readily available is unreliable: in general, people who are better at self-control instead reduce their exposure to temptation in the first place, and/or develop habits that make their intended behaviour more reliant on automatic processes than conscious control (Galla and Duckworth 2015; Angela L. Duckworth et al. 2016; Hofmann et al. 2012; Ent, Baumeister, and Tice 2015). Therefore, we should predict that digital devices, which make a wide range of behaviours constantly available with minimal effort, would come with significant self-control challenges and a corresponding need to translate people’s ordinary strategies for managing temptation into the digital realm.
This challenge is compounded by the business models of many tech companies, which incentive design that nudges people into using services frequently and extensively, a dynamic often referred to as the ‘attention economy’ (Davenport and Beck 2001; Einstein 2016; Wu 2016): in 1971, Herbert Simon noted that when information is overabundant, human attention becomes a scarce resource, which must be allocated efficiently among the information sources that may consume it (Simon 1971). Simon made this point in the context of system design, where he argued that digital systems should filter information to ensure the user is not distracted or overwhelmed by less relevant details. More recently, however, Simon’s insight has been applied to analyse how the explosion of readily available information via ICTs has led to a breakneck competition among technology and media companies over capturing and holding users’ attention (Harris 2016; Leslie 2016; Wu 2016). This competition has been intensified by the rise of ‘free’ as a dominant business model: if users do not pay for the product, such as in services provided by Facebook, Google, and a large majority of mobile apps (“Distribution of Free and Paid Android Apps 2019,” n.d.), companies usually monetise products through advertising, collection of user data (cf. ‘surveillance capitalism,’ Zuboff (2015)), or in-app purchases. This in turn creates a financial incentive to design in ways that keep users coming back to their digital devices and services and make them spend as much time as possible. (Perhaps unsurprisingly, the American author Nir Eyal’s book Hooked: How to Build Habit-Forming Products (2014), became an international best-seller.)
The incentives of the attention economy create a potential misalignment between how the end-user wishes to use a digital service (e.g., using Facebook for specific tasks such as event coordination or messaging) and how the product designer wishes the end-user to behave (e.g., spending as much time as possible on Facebook to optimise advertising revenue). Advertising agencies competing for attention is, of course, not a new development (Wu 2016). However, what is new — and why the challenge at this moment in history is particularly important to tackle — is that portable digital devices extend the battle for attention to any time of day and any situation, from the bathroom to the bedroom; can influence users in multi-modal and interactive formats; and can precisely target audiences using previously collected user data, at scale (Boyd and Crawford 2012; Fogg 2003).
2.1.4 The rise of digital self-control tools
In parallel with the massive investment of resources in keeping users ‘hooked’ on digital systems, online stores have seen the rise of a counter-movement in the form of a niche for ‘digital self-control tools’ (DSCTs). Thus, hundreds upon hundreds of apps and browser extensions now cater to people struggling with self-control over device use, and claim to provide means of resistance in the battle over their attention (Lyngs 2019d; Lyngs et al. 2019). As we will review in Chapter 3, these tools provide a wide range of interventions which may, e.g., block or remove digital distractions, track and visualise device use, remind users of their goals, or provide rewards and punishments for how devices are used. For example, they may restrict the amount of functionality available on devices’ home screen (such as the Android app LessPhone Launcher, Mohan (2019)) gamify self-control by tying device use to the wellbeing of virtual creatures (such as the smartphone app and browser extension Forest, Seekrtech (2018)), or hide or change content on distracting websites (such as the browser extension Newsfeed Eradicator, JDev (2019)). Some of these tools now have millions of users (Google Play (2017), cf. Chapter 4).
In 2018, the perceived demand for such tools, as well as public debate and pressure on tech platforms fanned by organisations including the Center for Humane Technology (formerly know as ‘Time Well Spent’), led Mark Zuckerberg to announce “making sure that time spent on Facebook is time well spent” as his annual personal challenge (Z. Mark 2018), and his company to announce tools for ‘managing your time on Facebook and Instagram’ (Ranadive and Ginsberg 2018); and Apple and Google to similarly implement features for visualising and limiting use on their operating systems (Apple 2018; Google 2018; Apple 2019). In 2019, public discussion has continued unabated, with a recent US senate hearing on the perceived threats of ‘Persuasive Technology’ (“Optimizing for Engagement: Understanding the Use of Persuasive Technology on Internet Platforms” 2019), and a call from a UK All-Party Parliamentary Group for a duty of care to be established on social media companies (All Party Parliamentary Group on Social Media and Young People’s Mental Health and Wellbeing 2019). Following the turning of the tide, Nir Eyal published a new book, this time called indistractable, focused on tools for managing attention and self-control when digital technologies are designed to hook their users in (Eyal 2019).
2.2 What does success look like?
If people struggle with distraction and self-control in relation to their use of digital devices, what are the criteria of success that design patterns should be benchmarked against? It is important to make our assumptions in this respect explicit, because they set the stage for what design patterns we imagine and how we evaluate effectiveness. Much recent debate, especially in relation to children and adolescents’ use of digital technology, has focused on ‘screen time’ and on how much of it might constitute unhealthy ‘overuse’ (Dickson et al. 2019; Przybylski and Weinstein 2017). Fittingly, a main focus of both Apple’s and Google’s new tools is to help people manage the overall amount of time they spend on their devices (Apple’s app is aptly named ‘Screen Time,’ “Use Screen Time on Your iPhone, iPad, or iPod Touch” (n.d.)) and find ‘the right balance’ (Google 2018). One underlying justification for this is the displacement hypothesis, according to which users should avoid excessive use of digital devices because it may supplant other important activities such as exercising, reading books, or socialising with peers and family (Neuman 1988; Przybylski and Weinstein 2017). Accordingly, researchers have tried to establish what amount of screen time might be ‘optimal’ to gain the benefits of digital connection without displacing other meaningful activities (Przybylski and Weinstein 2017; Przybylski, Orben, and Weinstein 2019).
The problem with this approach, however, is that today’s smartphones, tablets, and laptops support an incredible range of activities and contents, from social media, TV, and video streaming, to gaming, reading, writing, and shopping. Moreover, some platforms — such as Facebook — have themselves come to integrate a vast range of functionality. Therefore, whereas ‘screen time’ may be helpful as umbrella term, the ever-expanding range of digitally mediated activities makes it a poor indicator of whether people’s use aligns with what they intended (Cecchinato et al. 2019; cf. parallel discussions in research exploring relationships between screen time and well-being, Orben and Przybylski 2019b; Orben, Etchells, and Przybylski 2018). For example, one possible pitfall from using screen time to guide interventions is to get people to set simplified usage goals that are easy to enforce and measure (e.g., limit Facebook use to 30 minutes per day) but which fail to capture their actual goal (e.g., decrease time spent scrolling the newsfeed, but increase time spent in the Facebook group for their local Taekwondo club, Lukoff (2019); cf. research on targeted non-use Hiniker et al. (2016)).
For these reasons, several HCI researchers have argued that developing design patterns for helping people reduce ‘screen time’ is inadequate, and advocated for more contextual understandings (Hiniker et al. 2019; Lukoff 2019). However, what might that more specifically mean?
2.2.1 User behaviour vs ‘true intentions’ in HCI
In human-computer interaction research, the question of how to bridge the potential gap between what users actually do and what they ‘really wanted’ to do has a relatively long history. In the 1960’s, Warren Teitelman’s ‘Do What I Mean’ (DWIM) philosophy argued that systems should not just execute whatever potentially erroneous instructions users put into a terminal (Teitelman 1966). Instead, they should try to interpret users’ true intentions and correct their errors (the implication being DWIM, Now What I Say (or Do)). In practice, however, Teitelman’s error-correction systems were critiqued as merely reflecting what their designer would have meant (‘do what Teitelman means,’ Steele and Gabriel (1996)).
The issue has cropped up in more fundamental ways in the domains of decision support and recommender systems, where the gap is not just between what the user typed and what they really intended, but between recorded interaction behaviour and what can be inferred about the user’s wants and needs. In recent years, behaviourism has been the dominant paradigm for recommendation, expressed in trends such as measuring user activity in A/B tests instead of capturing user experiences in surveys and ethnographic analyses, and ignoring people’s explicit preferences when it disagrees with their behaviour (Ekstrand and Willemsen (2016); industry case studies include Facebook’s introduction of the newsfeed which involved striking contrast between users’ sentiment and their behaviour, Fisher (2018)). However, much recent critique of social media platforms has revolved around the problems associated with equating users’ true needs and desires with simple behavioural measures of engagement (cf. current debates about whether removing ‘like’ counts on social media platforms would benefit user well-being Martineau (2019)). Whereas people’s behaviour obviously provides some valuable information about their goals — on the basis of which an extensive knowledge base has been generated about how recommendations can effectively lead users to action — relying purely on behaviour may render recommender systems unable to distinguish between addiction and the user deriving value from the system (Ekstrand and Willemsen 2016). Thus, Ekstrand and Willemsen (2016) argued that in order to know whether users are satisfied with their choices both short-term and long-term; to know what keeps them from aligning their actions and desires if they are dissatisfied; and to know whether the recommender system is helping or hindering the user in achieving the goals they have for their life, explicit input from the user is necessary.
Numerous ways to explicitly elicit users’ preferences have been explored in the literature, including user ratings and example-critiquing in recommender systems (Pommeranz et al. 2012; Pu and Chen 2009), and pairwise comparison in decision support systems (Aloysius et al. 2006; L. Chen and Pu 2004). Moreover, pioneering work in this space explored ways to let users inspect and tweak a system’s model of them (Cook and Kay 1994; J. Kay 1997, 1995; Pu and Chen 2009). However, explicit methods have their own challenges. Foremost, the elicitation process itself influences what users say they want. For example, users may prefer different options based on whether they are framed as losses or gains (Pommeranz et al. 2012), or depending on the moment in time in which they are asked. What point in time reflects what the user ‘really’ wants - the most recent, a weighted average over the last day / week / year, or something else (Kahneman and Riis 2005; Redelmeier and Kahneman 1996)? Jameson et al. (2014) suggest that decision-support systems should optimise for outcomes that “the chooser is (or would be) satisfied with in retrospect, after having acquired the most relevant knowledge and experience,” but add that “Admittedly, this assumption is subject to debate…” (2014, 35).
Another point of tension is between broad or narrow construals of the user’s context. One of the foundational tenets of user-centred design, as articulated by Ritter, Baxter, and Churchill (2014), is to consider the user context more broadly. That is, to move beyond the immediate, task-related issues pertaining to a specific product, where the user’s goals can be more easily approximated, and instead view applications of technology as “the development of permanent support systems and not one-off products that are complete once implemented and deployed” (Ritter, Baxter, and Churchill 2014, 44). In other words, the designer should consider longer-term effects of systems on people’s lives, which in turn requires deeper insight in order to align systems with users’ more general goals, values, and life situation (cf. Peters, Calvo, and Ryan 2018). However, this runs the risk of not being actionable, partly because it involves a potentially boundless number of concerns in relation to a product’s impact, and partly because it leaves a much bigger role for normative disagreements over values and visions of the ‘good life’ and the role digital devices ‘ought’ to play, which are not easily resolved (cf. Orben 2019).
Summing up, Pommeranz et al. (2012) noted that “More research is needed to design preference elicitation interfaces that elicit correct preference information from the user” (2012, 361), and called for explicit consideration in HCI research of the normative aspects that might be required to determine such ‘correctness’ (cf. Lyngs et al. 2018).
2.2.2 The view from self-control research: Aligning device use with valued, longer term goals in the face of conflicting impulses
Whereas HCI research has run up against the challenge of distinguishing between what people do and what they ‘really want’ in relation to systems design, fundamental research on self-control within psychology, neuroscience, and behavioural economics have tackled this problem space for decades, with an aim to understand the mechanisms involved (Baumeister, Vohs, and Tice 2007; Hagger et al. 2010; Inzlicht, Schmeichel, and Macrae 2014; Kotabe and Hofmann 2015; Shea et al. 2014).
In contrast to notions of ‘rational actors’ with consistent preferences, this research has highlighted that people frequently experience internal conflict in which short-term desires (e.g., checking one’s Instagram feed) conflict with longer term goals that they, upon reflection, deem to be more valuable (e.g., doing well on a school test, Angela L. Duckworth and Steinberg (2015)). Often, people fail to act in accordance with their stated longer term goals (Consolvo, McDonald, and Landay 2009), which behavioural economists have described as ‘time-inconsistent preferences’ (Hoch and Loewenstein 1991; Ariely and Wertenbroch 2002) and explained through decision biases such as a tendency to value potential rewards less the further away they are in time (‘future discounting,’ Ainslie (2010); Critchfield and Kollins (2001)). For example, even though we may value getting a good night’s sleep and know that tomorrow we will appreciate having gone to bed at a reasonable hour, the short-term gratification of, e.g., watching another YouTube video often looms larger in the moment (see Ariely and Wertenbroch 2002).
Hence, this literature has defined ‘self-controlled behaviour’ as “actions aligned with valued, longer term goals in the face of conflicting impulses to seek immediate gratification” (Angela L. Duckworth and Steinberg 2015, 32). Sometimes, self-controlled behaviour involves inhibiting an undesired impulse (e.g., suppressing an urge to check one’s phone in a social situation) whereas it at other times involves strengthening a desired action (e.g., spending time on a MOOC course rather than on Facebook, cf. Fujita (2011); Angela L. Duckworth and Steinberg (2015)).
Research in this space has also investigated the many “tricks” by which people try to out outmanoeuvre their myopic present self, from distracting themselves from temptations in the heat of the moment, to ‘pre-committing’ to a particular course of action, or simply avoiding exposure to temptation (Angela L. Duckworth et al. 2016; Ariely and Wertenbroch 2002). Ultimately, all such strategies attempt to decrease the strength of momentarily rewarding, but ultimately less desired, impulses and/or increase the strength of enduringly beneficial, but relatively less immediately gratifying ones (Angela L. Duckworth et al. 2016).
Self-control has been studied under various names, including effortful control, will power, ego-resilience, and cognitive control and the literature on the topic is vast (see Angela L. Duckworth and Steinberg 2015; Inzlicht, Bartholow, and Hirsh 2015). For our purposes, the main take-away is that across research in this space, the notion of ‘success’ — what people ‘really want’ — is defined as being able to act in accordance with one’s enduringly valued goals in the face of conflicting urges that may be more potent in the moment (Angela L. Duckworth and Steinberg 2015). In chapter 3, we expand on one particular approach drawn from this literature, dual systems theory, which we then adapt to the context of self-control struggles in relation to digital device use, and apply throughout the rest of this thesis.
2.3 Studies of design patterns for digital self-control
When I began my DPhil project in the autumn of 2016, research into design patterns for supporting user self-control over digital device use was a relatively new area of exploration. Since then, research interest has rapidly grown. In this section, we review main design approaches, findings, and guiding theory from the studies conducted in this space. We add a brief overview of theory applied in the wider area of digital behaviour change interventions, before highlighting some of the limitations and opportunities given our current state of evidence.
Citation | Summary | Guiding conceptual framework | Evaluation | Control group? |
---|---|---|---|---|
Lottridge et al. (2012) | Firefox extension which classifies URLs as work or non-work, then makes non-work tabs less prominent and displays time spent | Multitasking, inhibitory brain function (Gazzaley et al. 2008) | experimental | no |
Löchtefeld, Böhmer, and Ganev (2013) | AppDetox, an Android app which let users voluntarily create rules intended to keep them from certain apps | None | observational | – |
Collins et al. (2014) | RescueTime, a commercial Windows/Mac application which provides visualisations of how much time is spent in different applications | Cognitive Load theory (Block, Hancock, and Zakay 2010) | experimental + observational | yes (in study 2) |
H. Lee et al. (2014) | SAMS, an Android app for tracking smartphone usage and setting time limits for app use | Relapse prevention model (Witkiewitz and Marlatt 2004), clinical guidelines for internet addiction (Kimberly S. Young 1999) | experimental | no |
Ko et al. (2015) | NUGU, a smartphone app which let users set goals for limiting usage, then share goals, contexts, and performance with friends and receive encouragement | Social Cognitive Theory (Bandura 1991) | experimental | no |
Foulonneau, Calvary, and Villain (2016) | TILT, an Android app which displays time spent and frequency of phone use over daily, weekly, and full history intervals, alongside about benefits of less use | Fogg’s behaviour model for persuasive design (Fogg 2003) | experimental | no |
Andone et al. (2016) | Menthal, a smartphone app displaying the ‘MScore,’ a single number summarising overall phone usage, as well as a series of main usage measures | None | none | – |
Hiniker et al. (2016) | MyTime, an Android app showing time spent in apps (and whether a daily limit was hit) plus a daily prompt asking what the user wished to achieve | None | experimental | no |
Y.-H. Kim et al. (2016) | TimeAware, an ambient Windows and Mac widget which presents time spent in ‘distracting’ or ‘productive’ applications | Framing effects (Marteau 1989) | experimental | no |
Ko et al. (2016) | Lock n’ LoL, a smartphone app which lets users as a group set their phones in a lock mode in which notifications are muted and usage restricted | None | observational | – |
Ruan et al. (2016) | PreventDark, an Android app which detects phone use in the dark and notifies the user that they should put it away | None | none | – |
Whittaker et al. (2016) | MeTime, a computer application providing a floating visualisation of time spent in different applications within the last 30 mins | None | experimental | no |
I. Kim et al. (2017) | Let’s FOCUS, an Android and iOS app letting users enter a ‘virtual room’ where notifications and apps are blocked; links to location or time | None | observational | – |
Park et al. (2017) | SCAN, a Android app which uses built-in sensors to detect opportune moments to deliver notifications so that they are minimally disruptive of social interaction | None | experimental | no |
J. Kim, Cho, and Lee (2017) | PomodoLock, a PC and Android application plus Chrome extension lets users set a timer for a fixed period during which distracting apps and websites are blocked across multiple devices | Strength model of self-control (Baumeister, Vohs, and Tice 2007) | experimental | no |
Marotta and Acquisti (2017) | Freedom, a commercial Windows/Mac/Android/iOS app which blocks access to distracting parts of the web or the internet altogether | Rational choice, commitment devices (Bryan, Karlan, and Nelson 2010) | experimental | yes |
Kovacs, Wu, and Bernstein (2018) | HabitLab, a Chrome extension in which the user sets time limit goals for specific sites, then tries a range of interventions to reach the goal | Numerous, including goal setting theory (Locke and Latham 2002), operant conditioning (Baron, Perone, and Galizio 1991), and self-consistency theory (Sherman 1980) | experimental | yes |
G. Mark, Czerwinski, and Iqbal (2018) | Freedom, described above | Attentional resources (Wickens 1980), Big 5 (McCrae and Costa 1999) | experimental | no |
Okeke et al. (2018) | Android app nudging users to close Facebook when a usage limit has been hit, using pulsing vibrations that stop when the user leaves the site | Nudge theory (Sunstein and Thaler 2008), operant conditioning (Iwata 1987) | experimental | yes |
Borghouts, Brumby, and Cox (2018) | A system (browser extension?) for providing people feedback on how long they navigate away from a task for | None | experimental | yes |
I. Kim, Lee, and Cha (2018) | A prototype smartphone app which automatically locks a user’s phone with a lock screen when the user has been stationery for more than 5 minutes (as this might indicate that the user is a ‘focusing context’), and displays how long it has been locked for | None | user study | – |
Kovacs et al. (2019) | HabitLab browser extension (described above) plus companion HabitLab Android app - similarly to the browser extension, in the app users specific target apps to reduce time spent. | Numerous, including goal setting theory (Locke and Latham 2002), operant conditioning (Baron, Perone, and Galizio 1991), and self-consistency theory (Sherman 1980) | experimental | yes |
Roffarello and De Russis (2019a) | Review of functionality + user reviews for 42 ‘digital wellbeing’ apps on Google Play; Socialize, an Android app providing usage statistics at the phone and app-level, and also can provide reminders or blocking after the phone or specific apps have been used for a certain amount of time. | None | review + experimental | no |
J. Kim, Jung, et al. (2019) | GoalKeeper, an Android app applying varying intensities of restrictive interventions (provide notifications, lock phone for increasing amounts of time, or lock phone for remainder of day) to keep users to their self-defined daily time limit for smartphone use. Also provides usage statistics. | Dual-self theory of impulse control (Fudenberg and Levine 2006), commitment devices (Bryan, Karlan, and Nelson 2010) | experimental | yes |
Tseng et al. (2019) | UpTime, a browser extension which senses activity and inactivity during computer work and automatically blocks distracting websites at points of transition; a Slack chatbot informs the user of the blocking and provides control if needed. | None | experimental | yes |
J. Kim, Park, et al. (2019) | Lockntype, an Android app requesting users to type in a fixed number of varying lengths (0 digits (“press OK to continue”), 10 digits, 30 digits) whenever a target app is launched | Expectancy-value theory (Rayburn and Palmgreen 1984), Uses and Gratifications theory (Wei and Lu 2014), dual systems-theory (J. St. B. T. Evans 2003) | experimental | yes |
Roffarello and De Russis (2019b) | Socialize (different to the identically named app above), an Android app for detecting and changing smartphone usage habits - when a habit is detected (e.g., using Facebook and Chrome between 10-12 AM,), the user can define an alternative behavior to be reminded about next time the habit is enacted. | Habit formation (Lally and Gardner 2013) | experimental | no |
van Velthoven, Powell, and Powell (2018) | Presents aim, main features, platform, and price for 21 tools for ‘regulating phone use’ identified by searching through https://alternativeto.net | None | review | – |
Biskjaer, Dalsgaard, and Halskov (2016) | Present a basic typology and preliminary framework for understanding features in ‘Do Not Disturb’ tools, on the basis of 10 select examples from the Apple App, Chrome Web, and Google Play stores as well as online technology magazines | None (develops their own typology) | review | – |
Table 2.1 summarises studies to date that have developed and/or evaluated design patterns for supporting user self-control of digital device use, along with their guiding self-regulation theory/s. I identified these studies by conducting searches on the ACM Digital Library, Web of Science, and Google Scholar using keywords including mobile app addiction, internet addiction, smartphone use, non-use, intentional use, intervention, self-regulation, self-control, and digital wellbeing, as well as by reviewing the references of listed studies published in 2018 or 2019. In line with the scoping considerations outlined in Chapter 1, I included studies which developed and/or evaluated new or existing design patterns, while excluding studies aimed at regulation of technology use in relation to children/families or distracted driving.
2.3.1 Tools/design patterns investigated
The existing studies have investigated a wide range of design patterns: Overall, some focus on visualisations of device use (e.g., Whittaker et al. (2016)’s MeTime displayed a floating window of relative time spent in computer applications over the past 30 minutes); others on blocking distractions (e.g., Marotta and Acquisti (2017) studied effects of using the commercial tool Freedom to block distracting websites among crowdworkers on Amazon’s platform Mechanical Turk); and yet others on goal setting (e.g., Ko et al. (2015)’s NUGU let users set goals for limiting smartphone use and share goals, contexts, and performance with friends).
Whereas earlier work mainly focused on one specific implementation of a potential design pattern, more recent investigations have moved towards more informative studies of (i) how varying key implementation parameters influences effectiveness, (ii) how interventions can be made more useful by being sensitive to context, and (iii) how cross-device use can be taken into account.
In relation to varying parameters, recent studies have varied amount of friction for accessing distraction instead of simply studying binary blocking: J. Kim, Jung, et al. (2019) compared effects of blocking target apps at three levels of intensity, and J. Kim, Park, et al. (2019) compared effects of requiring the user to type in digit combinations of varying lengths before target apps could be accessed.
In relation to context sensitivity, Park et al. (2017) investigated use of built-in smartphone sensors to detect socially appropriate moments to deliver notifications, and Tseng et al. (2019) studied how distraction blocking in work contexts might be made more useful by being automatically triggered at break-to-work transitions.
In relation to cross-device use, J. Kim, Cho, and Lee (2017) investigated benefits of coordinated distraction blocking on personal computers and smartphones, and Kovacs et al. (2019) evaluated potential ‘spillover’ effects from one device (or one distraction) to another, when applying interventions to reduce use of target apps or websites on smartphones and computers.
2.3.2 Evaluation approaches
In evaluating the effectiveness of such design patterns, most studies (20/29) have taken an experimental approach and compared user behaviour and perceptions when applying a particular intervention to its absence and/or some alternative intervention. Four studies have been purely observational and studied use and perceptions when, e.g., releasing a tool in online stores or deploying it as part of a student well-being campaign. Four studies have focused on (or included) reviews of available tools in online stores and described their features and/or created typologies of functionality. Two studies evaluated only whether the technical aspects of a proposed intervention worked as intended, but not how it influenced users’ behaviour or perceptions.
2.3.3 Findings
2.3.3.1 Behavioural effects
A basic outcome measured in most studies is time spent and frequency of use overall and/or in specific functionality such as social media. Overall, the existing studies suggest a strong potential for many of the tested strategies to influence these behavioural outcomes in targeted ways.
For example, Kovacs, Wu, and Bernstein (2018)‘s HabitLab, a Chrome extension and Android app which rotates between many interventions to discover what most effectively reduces users’ time spent (from removing newsfeeds to adding countdown timers), was found in controlled field experiments to reduce time spent on goal sites and apps (by 8% on the Chrome version, and 37% on the Android version in one of their studies). Moreover, Kovacs et al. (2019) found that reduced time on goal sites did not ‘spill over’ to increase time on other distractions or between devices.
Whereas the HabitLab investigations binned effects from a range of interventions, other studies point to effects of specific strategies. Thus, studies of distraction blocking suggest that this design patterns can reduce both overall device use and use of specific targeted functionality. For example, Tseng et al. (2019) found that when automatically blocking distracting websites during break-work transitions (users could override blocking via a chatbot), users visited distracting sites in 5.5% of transitions, compared to 14.8% without the system and 17.8% when blocking was self-initiated. Perhaps unsurprisingly, it has also been found that when distraction blocking is more difficult for the user to override, time spent is more effectively reduced, compared to ‘weaker’ solutions, though users generally prefer solutions that provide them some flexibility (J. Kim, Jung, et al. 2019). Adding ‘friction’ rather than blocking distractions per se has similarly been found to exert a potentially powerful influence on use: J. Kim, Park, et al. (2019) found that requiring users to type in a specific 30-digit sequence, before being allowed to use a target app, discouraged use in 48% of cases, compared to 27% for 10 digits and 13% for a pause-only version without number input.
The studies of visualising device use have found that this design pattern‘s potential to influence behaviour depends heavily on how visualisations are presented. Thus, one study of the commercial tool RescueTime found no impact on behaviour, which researchers attributed mainly to participants not engaging with the tool, as its visualisations needed to be actively accessed via a website (Collins et al. 2014). By contrast, Whittaker et al. (2016) found that their tool MeTime, which constantly displayed relative time in computer applications in a floating window, effectively reduced time spent in ’non-critical’ applications as well as overall time online. Y.-H. Kim et al. (2016)‘s evaluation of their similar tool TimeAware further found that influence on behaviour depended on how information is displayed –— a ’negative’ framing in which the widget highlighted time in ‘distracting’ applications increased users’ time in productive applications relative to total computer use, whereas a ‘positive’ framing which highlighted time spent in ‘productive’ applications had no influence on behaviour.
In terms of goal setting, the existing studies also suggest potential, depending on the implementation details. Hiniker et al.’s MyTime asked users to set a goal for daily time in target apps (with a “Time’s up!” dialog appearing when the limit is hit) and provided a daily prompt asking the user what they would like to achieve. This tool reduced overall daily phone use by 11% (33 minutes), via a selective reduction of time spent in apps users felt was a ‘waste of their time,’ while time in apps reported to be a ‘good use of their time’ was unaffected (Hiniker et al. 2016). Meanwhile, Ko et al. (2015) found that only the social version of their tool NUGU, in which users could share their goal for limiting smartphone use with others, significantly reduced usage; an alternative version, which involved only goal setting without the social accountability element, had no effect on behaviour.
2.3.3.2 Subjective effects
For the present thesis, the central question is how these design patterns affect users’ perception of the extent to which they are able to effectively use their devices in line with their goals. One complication is that the existing studies have conceptualised the problem space in somewhat different ways — from supporting productivity in professional or academic settings to improving social interactions — which means that an accordingly broad range of outcome measures have been used to assess user perceptions (from subjective workload to self-reported smartphone addiction). Nevertheless, combined results suggest that their tested interventions have the potential to positively influence users’ perceptions of their own device use in at least three directly relevant aspects: (i) increasing awareness of one’s patterns of device use, (ii) increasing perceived ability to focus on an intended task and be in control, (iii) preventing ‘chains of distraction.’
In terms of awareness of use, different types of interventions have been reported by research participants as having beneficial effects. For example, MeTime’s ambient window displaying relative usage in different applications (Whittaker et al. 2016) was reported by users to help them be more aware of how they used their computer and keep their usage goals in mind. Subjective reports have also pointed out that visualisations of use should be actionable for increased awareness to be beneficial. Thus, in Collins et al. (2014)‘s evaluation of RescueTime, participants reported not knowing what to do with the information obtained. Y.-H. Kim et al. (2016) suggested that their finding that an ambient display of time spent influenced behaviour only when time in ’distracting’ — as opposed to in ‘productive’ — applications were highlighted, could be explained by only the former being actionable to users.
Other types of interventions have also been reported to benefit awareness of use, especially in relation to raising awareness of use typically engaged in without conscious intent. For example, Okeke et al. (2018) made smartphones emit intermittent vibrations when a daily limit on Facebook use was hit, which was reported by participants to make them more aware of how they used the app. Similarly, a preliminary study by I. Kim, Lee, and Cha (2018) studied context-aware blocking on smartphones, in which a lock screen was displayed when a user was stationery for more than 5 minutes, and the user was asked to report purpose for use when unlocking the device. Participants said this intervention was particularly helpful for making them aware of the ways in which they often used their device without purpose.
In terms of perceived ability to focus and be in control during device use, benefits have in particular been reported in studies of distraction blocking. For example, most participants (74%) in the deployment of I. Kim et al. (2017)’s blocking app Let’s FOCUS for smartphones said the tool helped them focus better on class, and in G. Mark, Czerwinski, and Iqbal (2018)’s study of the blocking tool Freedom, participants who struggled with social media distractions significantly increased feeling of control over computer use with distractions blocked. J. Kim, Cho, and Lee (2017)’s study of PomodoLock provided a hint about underlying mechanisms, as participants reported that distraction blocking helped them be in control by alleviating the mental effort ordinarily needed to resist the temptation to check distracting functionality.
In terms of preventing chains of distraction, this benefit has been mentioned in several studies. For example, in G. Mark, Czerwinski, and Iqbal (2018)‘s study of Freedoom, participants explicitly said distraction blocking helped them avoid engaging in cascading distractions. Underlying this, Kovacs et al. (2019) noted that many apps and websites that users wish to reduce time on contain ’content aggregators’ (e.g., Facebook’s newsfeed) that point the user towards a multitude of other potential distractions, and so reducing time on target sites may therefore prevent cascading distraction and more broadly reduce time spent.
2.3.4 From one-size-fits-all to bespoke interventions
As more studies have been conducted, the findings from, especially, later studies have begun to unravel more specific questions related to when and for whom particular interventions are beneficial (cf. Klasnja et al. 2017). We have nowhere near enough evidence to answer such questions conclusively, but the existing findings do allow us to put together a preliminary picture of some of the considerations for design patterns that relate to (i) level of friction/user flexibility (where a fine balance needs to be struck), (ii) emotional state (where the usefulness of the same tool for the same user may vary over time), and (iii) individual differences (where, in particular, distraction blocking seems to be more useful for people who struggle more with distractions at the outset).
In relation to level of friction/user flexibility, the evolving evidence suggests that more intrusive strategies such as blocking need to strike a delicate balance. Some studies have found that when users need to self-initiate blocking of distractions, they simply do not engage with it and fail to benefit. For example, Marotta and Acquisti (2017) found that enforced blocking of Facebook and YouTube during working hours improved MTurk crowdworkers’ productivity and earnings, but when participants actively had to choose which websites to block and for how long, there was no effect. A version of J. Kim, Jung, et al. (2019)’s GoalKeeper, however, which strictly locked out users from target apps for the rest of the day after the daily limit was reached, seemed to be too restrictive and was experienced very negatively by many users, eliciting stress and anxiety, partly because it did not accommodate “out of routine” incidents where participants needed to use a particular app after being locked out. By contrast, Tseng et al. (2019)’s UpTime was viewed very positively by users –— and reduced self-reported stress –— despite applying automatic blocking of distractions, perhaps because users here could negotiate an override of the blocking via a chatbot. There may not be a simple answer to how blocking tools can strike the right design balance —– whereas participants in J. Kim, Jung, et al. (2019)’s study of GoalKeeper preferred a medium level of friction where they still retained some control, some also said they would find the strong-lockout version highly useful in specific situations where a particularly high level of focus is required, such as during exam periods.
In relation to emotional state, the evolving evidence suggests that this affects strategies’ usefulness, in somewhat complex ways. Thus, some participants using PomodoLock (J. Kim, Cho, and Lee 2017) to block distractions said they used the tool when they were ‘in a relaxed state without time pressure’ — when they were pressed for time, the tool was not necessary because the perceived external pressure already forced them into a state of concentration high enough to avoid self-interruptions (perhaps somewhat in contradiction to those of J. Kim, Jung, et al. (2019)’s participants who wanted strong-lockout during stressed exam periods). Similarly, J. Kim, Park, et al. (2019)’s study of varying levels of effort before target apps could be opened found that the effect of discouraged use was particularly strong when users were tired. More evidence needs to be collected, but findings here may usefully be compared to research from the self-control literature on how emotional states influence the ability to avoid temptation (e.g., Facebook users find it more difficult to self-regulate use of the network when in negative mood, Ryan et al. (2014); cf. Tice, Bratslavsky, and Baumeister (2001)).
Finally, recent studies have begun to investigate how design patterns’ effectiveness depends on individual difference. Thus, exploratory findings from studies of distraction blocking suggest the biggest benefits accrue to users who struggle the most with handling distractions at the outset: Mark et al. (2018) reported that those participants who experienced the greatest increase in focus with distractions blocked were those who self-reported as being more susceptible to social media distractions. Similarly, Kim et al. (2017) reported that the biggest productivity increase from using PomodoLock accrued to those who performed worst at baseline. Though an alternative interpretation in these two studies is a ‘ceiling’ effect — if one already scores high on some measure, there may be less room for improvement when an intervention is applied — J. Kim, Park, et al. (2019) found complementary evidence: whereas requiring participants to type in a greater number of digits before being allowed to used an app led to the greatest decline in use for most participants, there was a subgroup for whom a simple pause-only intervention achieved the same effect size. Thus, there may be some spectrum of individual differences that predict how intrusive design patterns should be to be effective.
2.3.5 Guiding theory
In this section, we make a few remarks about use of theory in HCI before providing an overview of the theory existing studies have applied to guide development and/or evaluation of interventions, as well as of theory applied in HCI research on behaviour change more generally. To pre-empt the findings, around half of the existing studies did not specify a guiding framework. Among those that do, a wide range of conceptual frameworks have been applied, and specific constructs are often presented as being instrumental to the development of an intervention. However, conceptual frameworks that explicitly address internal struggle between longer-term goals and conflicting impulses and habits — such as dual systems theories — have rarely been applied. More application of such frameworks has been called for by HCI researchers studying digital self-control as well as behaviour change more widely.
2.3.5.1 The role of theory
Research on design patterns for digital self-control represents a subset of HCI research on digital behaviour change interventions (Pinder et al. 2018). In HCI research on behaviour change, theory has been used for three main purposes (cf. Hekler et al. 2013): (i) to guide the design of digital systems, (ii) to guide evaluation of such systems, (iii) to define target users. Using theory for these purposes is not trivial, as theories’ level of specificity leave greater or lesser room for interpretation when using them to e.g. provide guidance on which functionality to support and how to implement it.
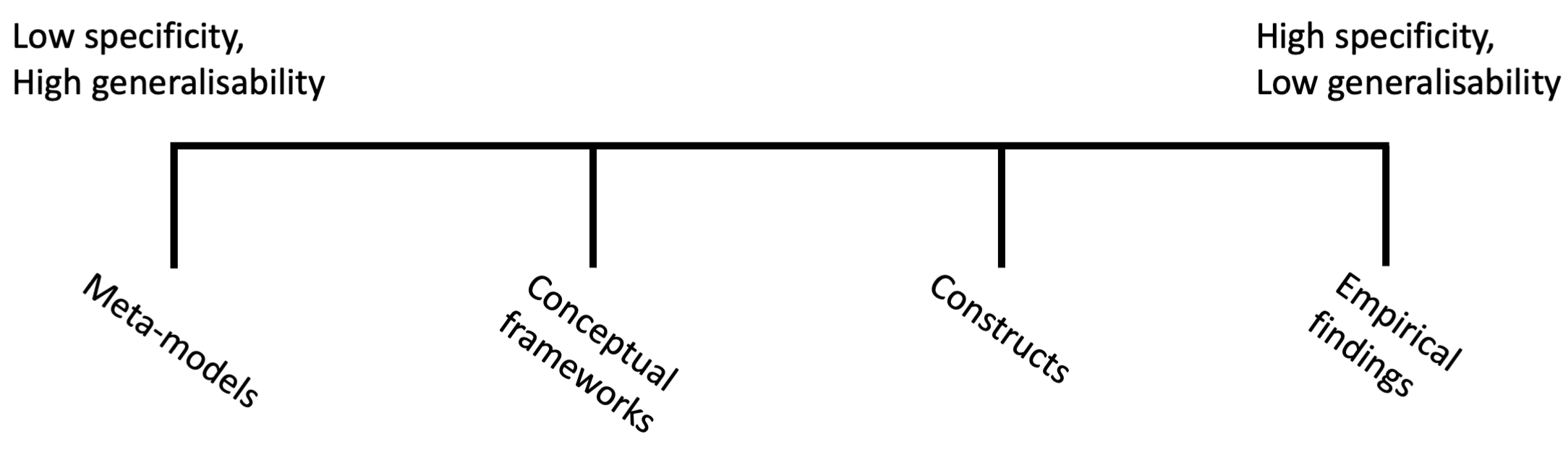
Figure 2.1: Spectrum of specificity in behavioural theories. Adapted from Hekler et al. (2013).
Hekler et al. (2013) suggest that behavioural theories reside on a spectrum of generality, from meta-models to empirical findings:
Meta-models represent the highest level of generality and are theories that organise multiple levels of influence on individuals’ behaviour.
For example, the ‘social ecological model’ (Sallis and Owen 1997) is a popular model in health-related behavioural science, which organises influences on behaviour into micro-level factors such as a person’s genetics, meso-level factors such as interpersonal relationships, and macro-level factors such as public policy and culture.
Meta-models are useful for identifying the ‘lens’ through which a researcher considers a given phenomenon in their application of more specific theories.
Conceptual frameworks zoom in on one or two levels of influence and provide a more specific account of how fundamental building blocks, or constructs, of a theory are interrelated.
Conceptual frameworks applied in existing work on design patterns for digital self-control include Social-Cognitive Theory (Bandura (1991); applied by Ko et al. (2015) in developing NUGU) and Prospect Theory (Kahneman and Tversky (1979); applied by Y.-H. Kim et al. (2016) in developing TimeAware).
Constructs are the basic mechanisms postulated by a conceptual framework as influencing behaviour.
For example, Social-Cognitive Theory postulates the construct of ‘self-efficacy’ — a person’s assessment of their ability to perform a given behaviour in a given context — as one of the key determinants of self-regulation.
Empirical findings reside at the lowest level, as some empirical work –— often ethnographic and other qualitative approaches — is carried out to produce concrete and contextually-specific data where previously developed theories are insufficient to guide research.
In turn, such data can provide a starting point for creation of new constructs and theories, allowing specific findings to be abstracted to create generalised knowledge (Hekler et al. 2013).
This spectrum may be useful as we consider the use of theory in previous studies, as it helps clarify which influences on behaviour are being excluded from a given conceptual framework.
2.3.5.2 Application of behavioural theory in existing studies of digital self-control design patterns
Table 2.1 includes the conceptual frameworks applied in existing studies. In 14 out of 29 studies, no conceptual framework was specified, with tool development and/or evaluation informed only by user-centered design methods such as surveys and interviews with target users and/or design experts, or (in the case of reviews of existing tools) bottom-up clustering of design features. The remaining studies referred to a wide range of frameworks drawn from psychology (e.g., Social-Cognitive Theory, classical conditioning, the strength model of self-control, dual systems theory), cognitive neuroscience (e.g., attentional resource theory, cognitive load theory, inhibitory brain function), (behavioural) economics (e.g., rational choice, expectancy-value theory, prospect theory), behaviour change (e.g., goal setting theory), and addiction research (e.g., the relapse prevention model). In some studies, constructs from these frameworks have directly informed design. For example, Y.-H. Kim et al. (2016)‘s comparison of effects of visualising time spent in ’productive’ vs. in ‘distracting’ applications drew on prospect theory’s construct of ‘loss aversion’ which describes differential sensitivity to gains and losses.
Applying a meta-model lens, we may observe that all conceptual frameworks applied in existing studies focus on the individual level. For example, even Social-Cognitive Theory, in which social influences on behaviour feature prominently in the form of social learning, views such influences through the lens of individual cognitive processes. To make sense of the challenge of digital self-control, we do need to keep in mind how larger dynamics in the attention economy incentivise design patterns which nudge users to behave in ways that are often in conflict with their personal usage goals (cf. section 2.1.3). However, since the focus of the present thesis is how to support individuals’ ability to exert self-control over device use, we similarly consider those dynamics in terms of how the resulting designs affect individuals. Therefore, the use of individual-level conceptual frameworks in existing studies aligns with the goals of the present thesis.
Within these individual-level frameworks, however, we may consider which aspects are included and which are left out. From the point of view of self-control research, the central phenomenon to be addressed is how designs may support users in resolving situations of conflict between momentary urges and more enduringly valued goals in line with the latter (Angela L. Duckworth and Steinberg 2015). Here, the empirical work in self-control research suggests that key components relate to interactions between different goals, impulses, and unconscious habits (Angela L. Duckworth et al. (2016); cf. Lukoff et al. (2018); Oulasvirta et al. (2012))
Considering the conceptual frameworks applied in existing research, however, most do not focus on interactions between habits and conscious intentions. For example, whereas Y.-H. Kim et al. (2016)’s use of framing effects from prospect theory was useful to their design of TimeAware, this framework provides a highly zoomed-out view of overall influence of gains and losses on behaviour that excludes underlying cognitive mechanisms. By contrast, G. Mark, Czerwinski, and Iqbal (2018) applied an Attentional Resource model (Wickens 1980) to explain why distraction blocking can be helpful (blocking frees up resources ordinarily used to attend to distractions), which provides a highly zoomed-in view of specific constraints on working memory capacity that excludes the bigger picture of goals and impulses.
Whereas these conceptual frameworks have proven useful for guiding intervention development and evaluation, a framework which explicitly includes the internal struggles described in self-control research might be useful for the purpose of considering the broader design space for design patterns for digital self-control. Among existing studies, only the dual systems framework used by J. Kim, Jung, et al. (2019) to frame the challenge addressed by their tool GoalKeeper provides this. Previous conceptual work in HCI on how ‘design frictions’ or ‘microboundaries’ may be used to support self-control over device use (Cox et al. 2016) have suggested that dual systems theory, which conceptualises human behaviour in terms of interactions between rapid, automatic ‘System 1’ processes and slower, more deliberate ‘System 2’ processes, could prove useful. J. Kim, Cho, and Lee (2017) referred to the ‘strength model of self-control’ in interpreting the results of their evaluation of PomodoLock, which has affinity with dual systems theory and postulates that the ability to inhibit impulses via conscious self-control fluctuates over time due to the depletion of a limited resource. However, in recent self-control research, the strength model is being replaced by motivational accounts based instead of fluctuations in the ‘expected value of control’ (see Chapter 3).
2.3.5.3 Theory in digital behaviour change interventions
A large body of HCI work exists on how digital tools can assist behaviour change in general (cf. Consolvo, McDonald, and Landay 2009; Epstein et al. 2015; Kersten-van Dijk et al. 2017; Yardley et al. 2016). A main focus within such research on ‘Digital Behaviour Change Interventions’ (DBCIs, Pinder et al. (2018)) is health, for example in relation to how digital interventions may help users exercise more (Consolvo, McDonald, and Landay 2009), quit smoking (Abroms et al. 2011; Heffner et al. 2015), eat more healthily (Coughlin et al. 2015), cope with stress (Gimpel, Regal, and Schmidt 2015), or manage chronic conditions (J. Wang et al. 2014).
Since digital self-control tools can be seen as a subset of DBCIs, which focuses specifically on behaviour change in relation to digital device use, application of theory within this research area is relevant to the present thesis. One recent review of 85 DBCI studies (Orji and Moffatt 2018) found the Transtheoretical Model (or Stages of Change, Prochaska, DiClemente, and Norcross (1993)) to be the most commonly referenced (13/85 papers), followed by Goal Setting Theory (Locke and Latham 2002) (5/85) and Social Conformity Theory (Asch 1955; Epley and Gilovich 1999) (3/85). 60% (51/85) did not specify any theoretical basis (cf. Schueller, Muñoz, and Mohr 2013; Wiafe and Nakata 2012), and none specified dual systems theory (cf. Pinder et al. 2018). The review also found that among studies which did specify underlying theories, most only mentioned them without explaining how the theoretical constructs informed the design and/or evaluation of actual intervention components (Orji and Moffatt 2018).
Another recent comprehensive review (Pinder et al. 2018) noted that most theories applied in DBCI research assume a rational, deliberative process as a key determinant of behaviour (e.g., the Transtheoretical Model, Prochaska, DiClemente, and Norcross (1993), or the Theory of Planned Behaviour, Ajzen (1991)). The authors argued (after extensive review and discussion) that dual systems theory could be well placed to guide DBCI research aimed at long-term behaviour change through breaking and forming habitual behaviour (Pinder et al. 2018; Stawarz, Cox, and Blandford 2015; Wood and Rünger 2016; cf. Webb et al. 2010).
2.4 Research gaps
In considering current limitations and research opportunities, we may keep advice from HCI work on behaviour change in mind: in assessing the state of HCI research on health behaviour change, Klasnja et al. (2017) pointed out that the goal is to generate usable evidence, that is, ‘empirical findings about the causal effects of behaviour change techniques and how those effects vary with individual differences, context of use, and system design.’ In other words, our findings should enable researchers, designers, and even end-users to make decisions about which design elements to include in new systems and how to implement them to maximise their potential to be effective for specific user groups. To generate such evidence, and allow for effective knowledge accumulation, studies should be carefully designed to allow us to infer whether observed changes in behaviour are due to an intervention of interest rather than other factors (Klasnja et al. 2017; cf. Cockburn, Gutwin, and Dix 2018; M. Kay, Nelson, and Hekler 2016; Orben 2019).
With this in mind, we highlight four areas of limitations and corresponding research opportunities motivating the research presented in this thesis.
2.4.1 (Theoretical) Exploring the usefulness of dual systems theory
As noted, frameworks which explicitly address interaction between conflicting goals, impulses, and habits have rarely been applied in existing studies. This is somewhat surprising, as such interactions are at the very core of self-control struggles. Among currently used frameworks, only J. Kim, Jung, et al. (2019)’s use of dual systems theory explicitly focuses on such interactions. Existing theoretical work on digital self-control (Cox et al. 2016) and digital behaviour change interventions (Pinder et al. 2018) have called for wider application of dual systems theory.
Chapter 3, 4, 5, and 6 of this thesis explore this research opportunity, by summarising a modern version of dual systems theory and applying it as a lens for organising, evaluating, and generating hypotheses in relation to design patterns for digital self-control.
2.4.2 (Empirical) Broad assessment of design patterns and implementations
Ideally, we want in hand clear evidence of the effects of representative examples of all plausible strategies and implementations across the entire design space of digital self-control interventions (J. Kim, Jung, et al. 2019; G. Mark, Czerwinski, and Iqbal 2018). The existing studies have made a good start, but some intervention ideas that are prominent among digital self-control tools in online stores (e.g., associating device use with the well-being of a virtual creature, as illustrated by Forest which has over 10 million users on Android alone, Seekrtech (2018)) have yet to be evaluted in HCI research. More generally, whereas controlled studies are appropriate and feasible for evaluating and comparing a small number of strategies, they are difficult to scale to broadly assess large numbers of strategies and implementations.
One opportunity for a complementary approach, which might help scope the range of strategies and implementations to explore in controlled studies, is to investigate the landscape of digital self-control tools on app and web stores. Here, widely available tools potentially represent hundreds of thousands of natural ‘micro-experiments’ (Daskalova 2018; J. Lee et al. 2017) in which individuals self-experiment with apps that represent not only one or more intervention strategies, but particular designs of those strategies. Other areas of HCI research have fruitfully taken this approach (e.g. research on mental health, Bakker et al. (2016); Lui, Marcus, and Barry (2017)), but it has so far been under-explored in relation to digital self-control. Chapters 3 and 4 explore this approach by analysing design features, user numbers, ratings, and reviews for a large sample of digital self-control tools on the Google Play, Chrome Web, and Apple App stores. Chapter 6 further explores how a broad sample of existing interventions can be used to elicit personal needs for such interventions in a workshop setting.
2.4.3 (Empirical) Understanding individual differences
Another research opportunity relates to understanding individual differences, where open questions include, e.g., whether some types of interventions are only effective for some people, and how widely people’s definitions of ‘distraction’ in relation to digital device use vary (G. Mark, Czerwinski, and Iqbal 2018; Cecchinato et al. 2019; Lukoff 2019). Existing research does not yet allow us to confidently answer such questions, and so additional collection of evidence is required to substantiate exploratory findings from intervention studies (e.g., G. Mark, Czerwinski, and Iqbal 2018; Hiniker et al. 2016; J. Kim, Cho, and Lee 2017) and ethnographic work (e.g., Lukoff et al. 2018; Tran et al. 2019).
Chapters 4, 5 and 6 contribute to this task: In Chapter 4, thematic analysis of user reviews for DSCTs in online stores contributes evidence on how people vary in the types of design patterns they seek; in Chapter 5, we draw out some of the individual variation emerging from, in particular, the semi-structured interviews with participants in a study of UI interventions for Facebook; and in Chapter 6, we present findings from workshops in which we investigated personal digital self-control struggles and used a card sorting task to elicit individual differences in preferences among potential interventions.
2.4.4 (Methodological) Improving evaluation to generate usable evidence
2.4.4.1 Ensuring experimental studies allow for causal inference
Following Klasnja et al. (2017)‘s assessment of HCI research for health behaviour change, we may consider the degree to which exiting studies allow us to infer which components of interventions for digital self-control are effective. To this effect, I examined whether existing studies using an experimental design (20 out of 29 studies) included control conditions in their study designs. That is, whether they compared the effects of an intervention not simply to its absence, but to some alternative intervention, which is important, because some effects result simply from how participants believe they are expected to behave (’demand characteristics,’ Nichols and Maner (2008)). Indeed, many of the outcomes we are most interested in (e.g., perceived control) may be highly sensitive to demand characteristics (‘come to think of it, I think I did feel more in control, now that you ask. Yes, I really did!’ Nichols and Maner (2008)). Moreover, asking participants to report on what components of an intervention made a difference to their behaviour is subject to biases and limitations of self-report (e.g., people may not remember clearly how they felt during specific moments of device use, some effects may occur outside of conscious awareness, etc., Gibbons (1983)).
We may try our best to infer causal components of an intervention by triangulating different types of data — for example, comparing evidence from behaviour, survey responses, and qualitative interviews (Mathison 1988; Munaf‘o and Davey Smith 2018). However, interpreting findings is much more straightforward when appropriate control conditions are included at the outset (cf. Klasnja et al. 2017).
Examining the designs of current studies, progress has been made from 2018 and later — just like there is a move away from proof-of-concept studies of a single interventions towards comparing different implementations of the same design pattern, study designs have become more carefully controlled. Thus, before 2018, only 1 out of 10 experimental studies (namely Marotta and Acquisti 2017) included a control condition in their study designs. These concerns are beginning to be addressed in studies from 2018 onwards, where 7 out of 10 experimental studies included control conditions.
Moreover, concerns have been reported over how the use of null-hypothesis significance testing (NHST) to infer effects of an intervention, in combination with a bias towards rejecting studies for publication that fail to report positive results, may lead experimenters to engage in problematic forms of ‘HARKing,’ or Hypothesising After the Results are Known (Kerr 1998). That is, researchers may conduct a large number of analyses and report only those that obtain significant results, without disclosing how many tests were run, while — consciously or unconsciously — reframing the experimental intention to match the outcome (Cockburn, Gutwin, and Dix 2018; Orben and Przybylski 2019a). Whereas exploratory research is essential to the scientific process, presenting results of exploratory data analyses as if they were confirmatory tests of an a priori hypothesis commits the ‘Texas sharp shooter’ fallacy (firing ones bullets and then drawing the target afterwards) and invalidates the ability of NHST to draw reliable and repeatable inferences (Simmons, Nelson, and Simonsohn 2011; Frankenhuis and Nettle 2018).
A simple remedy is to distinguish clearly between exploratory and confirmatory work — ideally through public pre-registration of planned analyses in advance of data collection and/or analysis (Cockburn, Gutwin, and Dix 2018). Another is to conduct replication studies, testing the reliability of previously published findings. Of the 20 experimental studies, 2 studies were explicitly exploratory (Roffarello and De Russis (2019a) and G. Mark, Czerwinski, and Iqbal (2018); the latter called for future confirmatory studies of their findings) while the remainder provided no explicit considerations of exploratory vs confirmatory findings. No studies referred to expected effect sizes as guiding their participant sample sizes, and none were replication studies (Tseng et al. (2019) did include a version of J. Kim, Cho, and Lee (2017)’s PomodoLock as a control condition in their study of UpTime, but this was a re-implementation that excluded core design features from the original study).
2.4.4.2 Open and transparent research
As called for in related research on digital well-being (Orben 2019), research on digital self-control may benefit from adopting open science practices: making materials, data, and analysis pipelines openly and transparently available can facilitate faster progress, reduce errors, and increase quality of research (cf. Schimmack 2019). In the case of digital self-control research, when considering the multitude of ways a design pattern may be implemented, knowledge accumulation between studies will be much improved if researchers are able to start out with the source code used by others rather than starting from scratch, as this may reduce noise from superficial implementation differences when comparing interventions. Only 1 (HabitLab, Kovacs (2019)) out of the 29 existing studies made materials, data and/or analysis scripts openly available.
In this thesis, Chapter 5’s mixed-methods study of interventions for self-control on Facebook attempts to improve on the methodology of existing studies by including a control condition and triangulating evidence from usage logging, survey responses, and qualitative interviews.
Chapter 3 and 5, which represent published work, make their materials, anonymised data, analysis pipelines, and manuscript source files (in R Markdown) available for other researchers via the Open Science Framework.
Finally, this thesis itself is written as a reproducible document using R Markdown (via the R package oxforddown
, Lyngs (2019b)), and its source files, supporting data, and materials are available via the Open Science Framework on osf.io/ed3wh/.
2.5 Summary
In this chapter, we have reviewed background literature relevant to this thesis’ investigation of how existing digital self-control tools can help us identify effective design patterns, grouped into four areas: (i) current evidence on the prevalence of self-control struggles in relation to digital device use, (ii) how to define the goal that design patterns for supporting self-control should strive to achieve, (iii) empirical insights generated by existing studies of specific interventions, (iv) theory applied in existing studies to guide development and/or evaluation of interventions. We then highlighted the limitations and opportunities that motivate the research presented in subsequent chapters.
In terms of evidence on struggles, a growing body of survey and qualitative evidence suggest a substantial proportion of people routinely struggle with exercising self-control over digital device use, with most recent studies focusing on smartphone use. Such struggles often takes the form of engaging in overly frequent check-ins with minimal awareness, or feeling ‘sucked in’ by one’s devices and using them for longer than intended. These struggles are reflected in a growing niche for ‘digital self-control tools’ on app and browser extensions stores which provide distraction blocking, visualisation tools, goal reminders, gamification, and other interventions meant to help people exercise self-control over device use. Increasing public debate on the topic recently led Apple, Google, and Facebook to begin incorporating similar ‘digital wellbeing’ features into their services. Two immediate causes of digital self-control struggles are that (i) smartphones and laptops make endless information, entertainment, and social connectivity available instantly and permanently, which existing psychological work on self-control suggests will come with substantial self-control challenges, and that (ii) the dynamics of the ‘attention economy’ incentivise companies to design services that nudge users into frequent and extensive use.
In terms of defining the goal of digital self-control strategies, several researchers have argued that simply helping people reduce ‘screen time’ is not helpful: as more and more activities become digitally mediated, screen time becomes a less and less useful indicator of whether people’s use aligns with their intentions. HCI research on decision support and recommender systems, which has tried to bridge the gap between what users ‘actually’ do and what they ‘really’ wanted, has found that relying purely on behavioural indicators to understand users’ intentions is problematic, but tended to avoid specifying what better alternatives look like. Standard definitions from fundamental research on self-control suggest that designing for digital self-control means to design in ways that help users align their device use with enduringly valued goals in the face of momentarily conflicting impulses and goals. Despite its shortcomings (which we discuss in Chapter 7), we adopt this as our working definition in the present thesis.
In terms of findings so far, current studies have established a solid potential for specific design patterns to assist people in aligning their device use with their longer-term goals. A range of approaches has been studied, mainly visualising use, goal setting and reminding, blocking access to distractions, or ‘nudging’ users by varying friction to distractions. The studies have found that such design patterns can significantly influence behaviour in a targeted way, and improve people’s awareness of their patterns of use, as well as their perceived ability to stay in control and maintain focus. Moreover, reducing time on sites and apps that people wish to spend less time have been found to not simply ‘spill over’ to other distractions. However, we are in the early days of collecting evidence on the potential impact across the design space of such interventions, the contexts in which they are useful, and the extent to which their effectiveness depends on individual difference.
In terms of application of theory, around half of existing studies did not specify any conceptual frameworks as guiding their work. Among those that do, a wide range of frameworks have been applied, with specific constructs often presented as instrumental to the development of an intervention. However, frameworks that explicitly address internal struggle between longer-term goals and conflicting impulses and habits have rarely been applied. HCI researchers working on digital self-control, as well as on behaviour change more widely, have suggested that dual systems theory — which only one existing study explicitly referred to — might be useful in future research as a framework which readily address conflicting goals, habits, and impulses.
On the basis of this review, we highlighted four areas of current limitations (and corresponding research opportunities) which motivate the thesis research:
(Theoretical) Exploring the utility of dual system theory
Even though interactions between conflicting goals, impulses, and habits are at the core of self-control struggles, conceptual frameworks that explicitly address such interactions have rarely been applied.
Chapter 3 summarises a modern version of dual systems theory, and Chapter 3, 4, 5, and 6 apply it as a lens for organising, evaluating, and generating hypotheses for design patterns.(Empirical) Broadly assessing possible design patterns and implementations
The design space for digital self-control interventions is vast, but controlled studies are difficult to scale to broadly assess large numbers of design patterns and implementations.
Chapter 3 and 4 explore a complementary approach: investigating the landscape of DSCTs on app and web stores, which represent hundreds of thousands of natural ‘micro-experiments.’ Chapter 6 further uses a broad sample of existing interventions to elicit personal needs for such interventions in a workshop setting.(Empirical) Investigating individual differences
A few studies suggest the effectiveness of their tested strategies is contingent on personality differences, but more evidence is needed to assess how reliable the initial findings are, and how much people’s definitions of ‘distraction’ vary in the first place.
Chapter 4 contributes evidence via thematic analysis of user reviews of digital self-control tools in online stores, Chapter 5 presents evidence from semi-structured interviews in a study of UI interventions for Facebook, and Chapter 6 presents results from four ‘Reducing Digital Distraction’ workshops which includes elicitation of individual preferences among existing interventions in a card sorting task.(Methodological) Improving evaluations to generate usable evidence
There is room for improvement in relation to making evaluation studies robust and embracing open science practices.
Chapter 3 and 5, which represent published work, make materials, data, analysis pipelines, and manuscript source files openly available. Moreover, this thesis’ source files, supporting data, and materials are available as a repository on the Open Science Framework, osf.io/ed3wh.