7 Discussion
This thesis set out to examine how existing digital self-control tools can help us identify effective design patterns for supporting self-control over digital device use. How have our resulting investigations of the landscape of DSCTs in online stores, UI interventions for self-control on Facebook, workshops on digital distraction with university students, and the use of dual systems theory as a guide throughout, helped us answer this question? In this discussion chapter, we first summarise the findings from our investigations. We then reflect on some of the challenges in relation to methodology and application of theory. Afterwards, we revisit what ‘success’ for design patterns for digital self-control might look like, recommending that our primary concern should be to empower users to sculpt their digital environments such that the amount and motivational pull of the information they are exposed to match their abilities and limitations. Finally, we consider a longer-term vision for the Reducing Digital Distraction Workshop as a tool for future research and practical impact.
7.1 Overview of results
As outlined in Chapter 2, the research presented in this thesis was motivated by current limitations and opportunities for digital self-control research in four areas: (i) a lack of application of conceptual frameworks that explicitly address interactions between conflicting goals and impulses, (ii) challenges around scaling controlled studies to large numbers of design patterns and implementations, (iii) a need for additional evidence on individual differences, (iv) a need for adoption of more robust, open, and transparent research practices.
Chapter 3 served two purposes: to introduce a dual systems framework and its potential usefulness for digital self-control research; and to characterise the design space for design patterns by analysing features in existing DSCTs in online stores. In introducing the dual systems framework, we extended existing applications in HCI with the concept of ‘expected value of control’ (EVC), which is considered central in the recent neuroscience literature as an explanation of why the ability to exercise self-control fluctuates over time and with emotional state. As a mediator of the strength of conscious self-control, EVC demystifies self-control and helps clarify how specific design features may work to scaffold it. In analysing DSCTs in online stores, we identified widely used and theoretically interesting design ideas under-explored in HCI research, such as tying device use to the well-being of a virtual creature. By applying the dual systems framework to the feature analysis, we also identified cognitive mechanisms under-explored in current tools, such as sensitivity to delay.
Whereas Chapter 3 analysed only the functionality of current tools, Chapter 4 added analysis of user numbers, average ratings, and reviews, which provide indicators of the outcomes of users’ micro-experiments with these tools. Our analysis of the content of user reviews corroborated findings from previous research: DSCTs help people focus on important, but effortful, tasks in the face of readily available digital distractions. Moreover, users search for tools which provide a level of friction or reward that is ‘just right’ to overcome temptation without being overly annoying, but there is widespread variation among users on where this ‘Goldilocks’ level is. Our analysis of user numbers and ratings generated new findings and hypotheses: for example, tools which combine more than one type of design pattern receive higher ratings, which might suggest that targeting different psychological mechanisms is a more effective way to provide enough incentive to cause behaviour change without being annoying, compared to ramping up the intensity of a single mechanism. In combination, Chapters 3 and 4 provided the first larger-scale, rigorous investigation of the online landscape of DSCTs as a rich resource for digital self-control research.
We can use such investigations to scope the range of design patterns and implementations to explore in controlled studies. Chapter 5 presented a controlled study informed in this way: surveying the landscape of DSCTs showed that a sizeable number of tools target Facebook in the form of browser extensions that, e.g., remove the newsfeed, but no existing studies have investigated the potential of these interventions to help users struggling with self-control. Chapter 5‘s study demonstrated that two such interventions — goal reminders and removing the newsfeed — can potently influence behaviour and reduce unintended use, suggesting this as a fruitful avenue for research into remedies for problematic use that present a less tall barrier to action than deactivating or deleting one’s account. Post-study interviews contributed qualitative evidence on more complex questions explored in recent studies of design patterns for digital self-control, such as how to accommodate individual differences in personalities and self-control needs, and how to avoid ’backfire’ effects where users rebel against intrusive interventions rather than being helped by them.
Finally, Chapter 6 explored how we may use the landscape of existing tools to elicit personal needs for digital self-control interventions in specific populations: in a workshop format developed with the University of Oxford Counselling Service, students reflected on their struggles and goals, explored a broad sample of solution interventions drawn from previous chapters in this thesis, and committed to trying out their favourite options. Their preferences varied, but interventions that targeted specific distracting elements were found to be especially useful (e.g., removing Facebook’s newsfeed). Hinting at avenues for future research, participants also wished for tools that could serve as `training wheels’ for improving self-discipline over time, i.e., that they could use as external support initially but which would later allow them to control themselves better in their absence.
7.2 Reflections on methodology
7.2.1 Challenges of defining the problem
As we saw in Chapter 2, it is challenging to precisely demarcate the problem that overlapping HCI research on ‘addictive use,’ ‘problematic use,’ ‘non-use,’ and ‘digital wellbeing’ is seeking to address. In this thesis, we drew on basic self-control research and defined ‘digital self-control’ as the ability to align digital device use with one’s enduringly valued goals in the face of momentarily conflicting goals and impulses. This definition applies an individual lens and suggests, for example, that spending an excessive amount of time playing video games is problematic only to the extent that it conflicts with one’s longer-term goals (Lyngs 2019c).
This approach has limitations. First, effects of ‘persuasive designs’ are currently much discussed in relation to children and adolescents. For populations less capable of assessing the longer-term impact of their actions, focusing solely on people’s self-perceived ability to align their device use with their enduringly valued goals is likely too narrow an approach. Though I would argue that focusing on individual self-control is the most practical approach when considering functional, adult users, when we consider individuals still undergoing developmental maturation, we cannot avoid normative discussions about which patterns of device use are more likely to nurture or harm human flourishing. Here, high-quality research on the relationship between particular notions of well-being, device use, and design patterns is necessary to guide parents and decision-makers (Hiniker et al. 2019). In this thesis, we narrowed the focus of our investigations to design patterns for supporting self-control among functional, adult users, because the ability of adults to effectively align their device use with their longer-term goals should, arguably, be an uncontroversial minimum goal when ‘designing for digital well-being’ (Lyngs et al. 2018).12 Such a focus allows us to make research progress without getting caught up from the outset in disagreements over what constitutes a ‘good life’ and what role digital devices ‘ought’ to play in this respect (cf. Orben 2019). Moreover, experience sampling studies suggest that the ability to exert self-control mediates effects of media use (including ICTs) on well-being (Hofmann, Reinecke, and Meier 2016), which provides additional reason to take digital self-control as our starting point.
A second limitation of our approach is that the meaning of ‘enduringly valued’ or ‘longer-term’ goals is not clearly defined (cf. parallel discussions between ‘proximate’ and ‘distal’ goals in HCI research on behaviour change, Klasnja et al. (2017)). In the self-control literature, its meaning tends to simply be illustrated through examples of prototypical self-control failure, such as giving into a momentary temptation to eat chocolate cake when on a diet, or procrastinating by scrolling through Instagram instead of writing the essay one is at the library to finish (cf. Angela L. Duckworth et al. 2016). However, just how long or how highly a goal should be valued to be considered ‘longer term’ or ‘enduringly’ valued is not clearly specified. Therefore, implications for, e.g., how designers can elicit users’ ‘true’ preferences are widely open to interpretation (cf. Lyngs et al. 2018). For example, imagine a university student watching her favourite TV show on Netflix. It is getting late, and she knows that she ought to go to bed, but feels tempted to watch one more episode. She may even take a moment to reflect and conclude that, on balance, watching another episode is more valuable to her than getting her regular hours of sleep, before clicking ‘play.’ However, in the morning she feels exhausted and regrets her decision from the night before. Or imagine she remains happy with her decision in the morning, and only months later realises that a consistent pattern of nightly Netflix watching seriously harmed her longer-term goals because she often missed morning lectures and ended up getting a bad grade. Which time span of reflection and valuation should we design to support? Moreover, people might have a sense in the moment of whether they are focusing on a valued shorter-term goal (e.g., focusing on that work task one needs to complete; or being present and attentive with one’s friends on a night out), but differ in how clearly they set longer-term goals and how consistently they hold them (cf. Angela L. Duckworth et al. 2007).
In considering these questions, it is important that we do not let the perfect become the enemy of the good. Whereas real-life examples tend to be more ambiguous than the ‘easy’ case, in which people’s in-the-moment and retrospective judgment are in perfect agreement, discussing how to resolve these tensions gets us asking the right questions, compared to the attention economy’s status quo of equating ‘enduringly valued goals’ with ‘behaviour which optimises ad revenue or data collection.’ Thus, a somewhat vague definition will still do if it encourages the right line of thinking — as an example from industry, Nir Eyal proposed a ‘regret test’ as a practical guideline for designers to consider what “users do and don’t want,” and act in an ethically responsible manner:
If people knew everything the product designer knows, would they still execute the intended behavior? Are they likely to regret doing this? If users would regret taking the action, the technique fails the regret test and shouldn’t be built into the product, because it manipulated people into doing something they didn’t want to do.
In so far as people regret actions because they diverge from their enduringly valued goals, this approach is similar to our conceptualisation of digital self-control. Whereas there is wide room for interpretation around what methods might be favourable for eliciting ‘correct’ preferences, a simple option to explore in future research could be for systems simply to allow users to choose between possible ways to infer their ‘enduringly valued goals’ (cf. Lyngs et al. 2018; Harambam et al. 2019).
7.2.2 Challenges of measurement
How we define the problem in turn has direct implications for what and when to measure in evaluation studies, which come with its own challenges.
What to measure
As we saw in Chapter 2, existing studies have — depending on how researchers have framed the problem — applied measurement instruments ranging from perceived workload to subjective smartphone addiction. When focusing on the problem through the lens of self-control, the most relevant measurements relate to self-perceived ability to align usage with one’s goals. This in turn prompts considerations around how broadly goal alignment should be construed: At one end of the spectrum, researchers may assess immediate goals for use of a specific service (e.g., in Chapter 5, participants in the goal reminder condition were prompted to type in their immediate usage goals for Facebook use) and subsequently assess whether they effectively achieved that goal (cf. Angela L. Duckworth et al. 2016). At the other end of the spectrum, researchers may assess a global sense of whether people feel able to align their use of digital devices with their longer-term goals (e.g., Ko et al. (2015) and Roffarello and De Russis (2019a) adapted the General Self-Efficacy Scale, cf. Luszczynska, Scholz, and Schwarzer (2005), to the context of self-regulation of smartphone use). This methodological question has no ‘correct’ answer, but researchers should clearly specify their choice and discuss the theoretical implications. In our study of interventions for self-control on Facebook, we relied mainly on self-report measures of overall usage patterns and perceived control, drawn from existing research on Problematic Facebook Use and distraction blocking (G. Mark, Czerwinski, and Iqbal 2018). We made this choice to allow our findings to be compared directly with findings from previous work, but follow-up research may explore ways to more directly assess people’s sense of using Facebook in accordance with their goals.
Moreover, most studies have focused on one device only, even though digital self-control struggles in the real world often involves cross-device use. This prompts similar questions over how to assess people’s sense of their ability to use their combined devices in line with their goals. Only two existing studies of design patterns tackled cross-device interactions (J. Kim, Cho, and Lee 2017; Kovacs et al. 2019), and so there is a need for further exploration of both effective design patterns and effective measurement approaches for assessing the impact on perceived self-control ability in multi-device contexts.
When to measure
In accordance with the challenges of defining the problem — and similar to concerns in research on ‘screen time’ and well-being (Orben 2019) — researchers should clearly specify the time frame at which they measure effects of interest, and discuss the theoretical implications. At one end of the spectrum, people’s in-the-moment reports of whether they are using their devices in line with their goals could be assessed using experience sampling methods. This approach has proven useful in studies of media use and self-control (Reinecke and Hofmann 2016) and is starting to be adopted in research on screen time and well-being (Johannes et al. 2019), as well as experience of ‘meaningfulness’ during smartphone use (Lukoff et al. 2018), but has yet to be applied in evaluations of design patterns for digital self-control. Thus, existing studies have focused on retrospective assessment at various time points, e.g., by the end of each day (e.g., G. Mark, Czerwinski, and Iqbal 2018), or in post-study interviews (e.g., Whittaker et al. 2016). This thesis’ controlled study (Chapter 5) also used retrospective assessment, via surveys after each 2-week study block, and post-study interviews. To help resolve theoretical and empirical questions, future digital self-control research will benefit from using experience-sampling methods to study how in-the-moment assessment compares to retrospective assessment of self-controlled device use, and how this is influenced by design patterns to support self-control.
How to measure
As the self-control lens implies subjective judgment, careful consideration of how elicitation methods influence self-report is essential. We previously touched on some of the challenges in this respect (see section 2.4.4.1), such as memory biases (Kahneman and Riis 2005), inherent limits of introspection (Gibbons 1983), and demand characteristics (Nichols and Maner 2008). In Chapter 5‘s controlled study, we tried to address this challenge by collecting and triangulating multiple types of data, namely logging of actual behaviour, bi-weekly surveys, and semi-structured interviews, and including a control condition. Whereas this provides no methodological silver bullet, control conditions help us interpret self-report data by providing a means to assess how the study process itself influences self-report (e.g., we observed that survey scores on ’overuse’ changed over time in all conditions, including in the control condition), and triangulating different data sources allow us to assess how self-report relates to measured behaviour.
7.2.3 Generating usable evidence through robust and open science
Digital self-control research should aim to generate ‘usable evidence,’ that is, insight into causal effects of specific design patterns, and how such effects vary with implementation details, contexts of use, and individual differences (cf. Klasnja et al. (2017), see section 2.4). As outlined in section 2.4.4, this aim will be furthered by carefully designing experimental studies that include control conditions so that the appropriate comparisons can be made in the first place, as well as by following the current push in related disciplines for more robust and open research practices (cf. Haroz 2019). In digital self-control research to date, experimental methodology has matured in studies from 2018 onwards, but adoption of open science practices is lagging behind. To realise the potential of the existing studies, and build on it in ways that lead to robust, usable evidence, open science practices are key. Here, we reiterate the importance of open sharing of materials, data13, and analysis scripts:
First, as mentioned in section 2.4.4.2, open science allows us to more effectively accumulate knowledge between studies, partly because starting from others’ materials when replicating or extending previous studies helps reduce noise from implementation and analysis differences. This was a real concern for some of the work in this thesis. For example, Chapter 5‘s study of self-control on Facebook followed Y. Wang and Mark (2018) in using the ROSE browser extension (Poller 2019) to log participants’ use on their laptops. When analysing participant data collected with this extension, we had to make a large number of data processing decisions, from thresholds for excluding data points likely to be erroneous, to precisely how time spent should be calculated. Such analytical decisions amount to a rapidly growing ‘garden of forking paths,’ where the results presented in the final paper show only one specific path (Orben and Przybylski 2019a)14. Directly comparing our findings to those of Y. Wang and Mark (2018) required the exact detail of how they analysed their data, beyond the broader verbal description of the data wrangling provided in their paper. However, neither materials, data, or analysis scripts were available with their paper, and neither of the authors responded to repeated email inquiries.
A second motivation for open science practices in this research area is that the research space itself can be approached in many different ways, as discussed in the previous sections. Therefore, it is important that we make our materials and data openly and transparently available to others, because this will allow us to better reuse and reinterpret previous findings as we make progress on theoretical questions.
In this thesis, we attempted to follow open science practices throughout, by making materials, data and analysis pipelines openly available and writing the manuscripts as reproducible, plain text documents using R Markdown (Xie, Allaire, and Grolemund 2018).
7.3 Reflections on use of theory
7.3.1 Alternatives to dual systems theory
This thesis relied on the dual systems framework introduced in Chapter 3 to categorise and evaluate design patterns, interpret findings, and suggest new opportunities, in line with recent HCI research on digital self-control and behaviour change more widely (Cox et al. 2016; Pinder et al. 2018). This had some advantages: First, this framework explicitly addresses interactions between conscious goals and automatic habits and impulses, which are at the core of self-control struggles, and which HCI research on behaviour change has argued are key for guiding interventions aimed at long-term change (Pinder et al. 2018). Second, the framework readily connects to ongoing research on self-regulation in the cognitive neurosciences, as illustrated by Chapter 3‘s extension of previous HCI use of the framework with recent research on the ’expected value of control.’ Therefore, the framework may be unpacked into more granular theories of, e.g., different constructs involved in processing of rewards, if design guidance from more specific constructs is called for (cf. Klasnja et al. 2017). In this way, the dual systems approach may both at a high level capture the main psychological mechanisms involved in digital self-control, and provide more detailed theoretical pointers, which gives the framework substantial mileage.
This is not to say that that the dual systems framework is necessarily ‘better’ than what has been applied in existing studies. Different frameworks direct attention and research efforts in different ways that can be more or less appropriate for a given research question, and existing studies have amply demonstrated that specific constructs drawn from other frameworks can guide effective design. However, for the purposes of the present thesis, the dual systems framework was particularly useful, because it provided a more inclusive view well suited to the initial chapters’ broad characterisation of the design space. In our further investigations of the framework’s potential in the controlled study of Facebook use, it also proved useful for guiding selection of specific interventions among many, and for generating hypotheses about potential and limitations to be explored in future research.
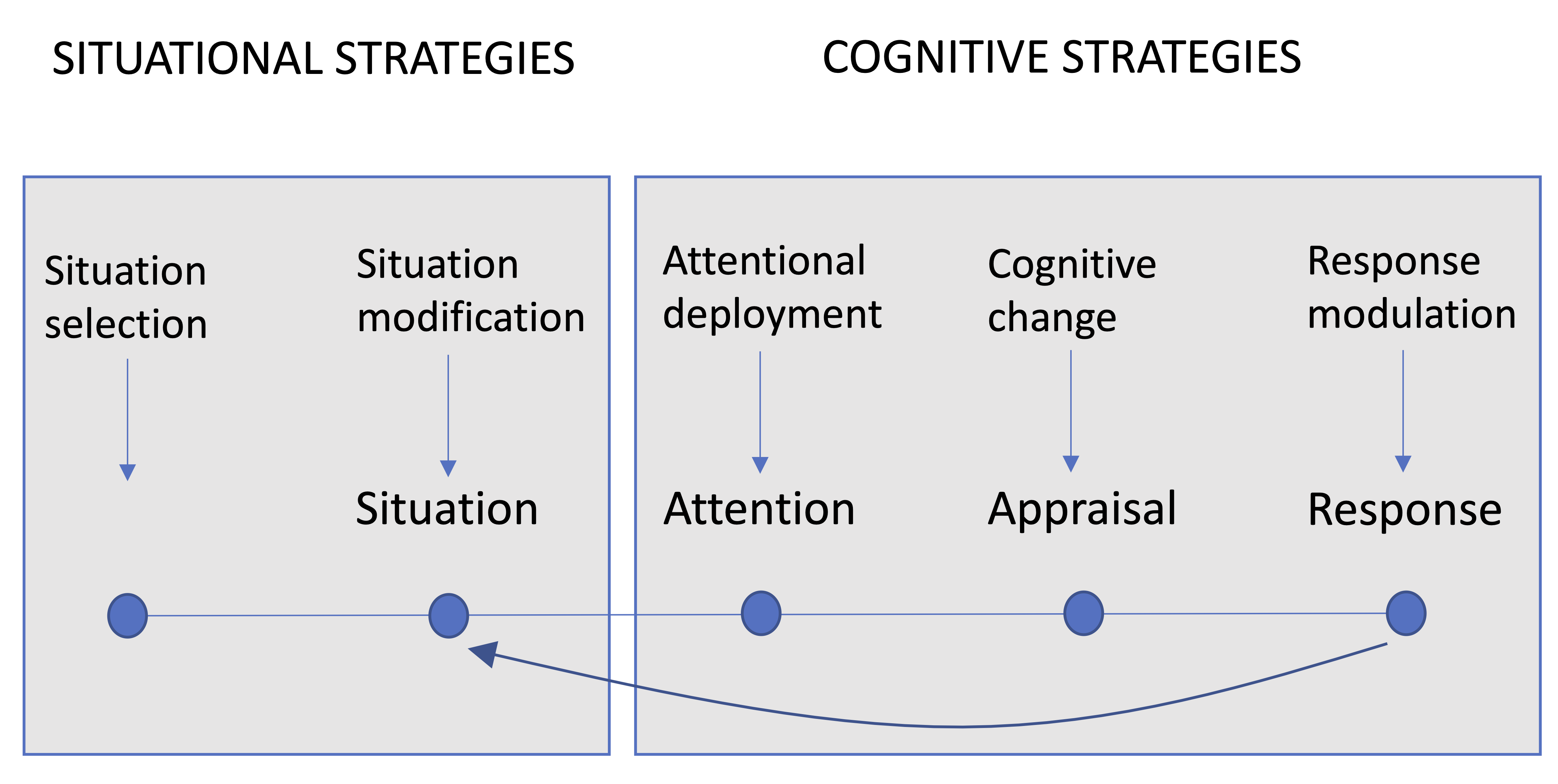
Figure 7.1: The process model of self-control (adapted from Angela L. Duckworth et al. (2016)). The framework focuses on how ‘impulses’ — responce tendencies to think, feel, or act — develop over time, and organises self-control strategies according to the stage of impulse generation at which they intervene.
There may be further room to explore conceptual frameworks popular in psychological research on self-control but rarely applied in HCI. Currently, one of the most influential frameworks of self-control in psychology is the ‘process model’ (Angela L. Duckworth, Gendler, and Gross 2014), adapted from James J. Gross’ work on emotion regulation (Gross 2015). This framework focuses on how ‘impulses’ — response tendencies to think, feel, or act — develop over time, and organises people’s self-control strategies according to the stage of impulse generation at which they intervene (see Figure 7.1). In this framework, self-control strategies work either by choosing what situations to expose oneself to (situation selection); changing the circumstances of the situation one is in (situation modification); changing what one pays attention to within a given situation (attentional deployment); re-adjusting how one values what one does pay attention to (cognitive change); or by directly inhibiting or enhancing impulses (response modulation). This model has been used by Angela L. Duckworth et al. (2016) to study self-control strategies among university students, has been preliminary applied in HCI work on emotion regulation (Miri et al. 2018), and may prove useful in future digital self-control research.15
Other work from basic self-control research that may supply avenues of inspiration includes research on how people’s assessment of the opportunity costs of their current task influences mindwandering, boredom, and mental effort (Forrin, Risko, and Smilek 2019; Kurzban et al. 2013). This research may be useful for understanding effects of how digital devices, such as smartphones, introduce additional behavioural options to their users at each time step (cf. Dora et al. 2019; Lyngs 2017a), and has affinity with long-standing HCI research on ‘information foraging’ (Pirolli and Card 1995).
7.3.2 Understanding individual differences
Understanding what interventions work better for whom is key to generating usable evidence. The studies so far are beginning to paint a picture of substantial individual variation — with light-touch goal reminders sufficient to scaffold self-control for some, and high-friction distraction blocking needed by others — and of how such variation might be predicted by measures such as Big Five personality traits or susceptibility to social media distractions.
Psychological theory may be helpful to advance our understanding in this respect, in so far that it indicates how individual differences map to relative strengths and weaknesses in specific self-regulation mechanisms, which in turn may suggest particular types of interventions as more relevant. The dual systems framework may also be valuable for this purpose: for example, the sensitivity to delay that forms part of the Expected Value of Control (cf. 3.2.5) is closely related to measures of ‘impulsivity’ (Steel and König 2006). Therefore, design patterns focusing on this component (e.g., increasing loading times of distracting websites) may be more effective for individuals scoring high on impulsivity. Similarly, the dual systems framework suggests that measures of relative working memory capacity would predict individual differences in the effectiveness of interventions which reduce the amount of potentially distracting information the user is exposed to, or which use goal reminders to keep a focal task from being forgotten (cf. section 5.6.1).
Zooming out, our understanding of individual differences is also likely to be helped by drawing on theories that apply a wider socioeconomic lens (cf. section 2.3.5.1). Thus, sociological research has found that self-control ability predicts many valued life outcomes, including physical health, substance dependence, personal finances, and criminal offences (Cheung et al. 2014; Moffitt et al. 2011). Accordingly, interventions addressing self-control (e.g., improving the degree of control that people perceive they have over their own lives and their environment, Gillebaart and Ridder (2019)) have been suggested as central to reducing societal problems (Moffitt et al. 2011). We may therefore expect socioeconomic concerns to be relevant for digital self-control research (cf. Kaba and Meso 2019): first, baseline variation in self-control ability, which correlates with indicators of socioeconomic status, is likely to translate into similar variation in digital self-control struggles. Second, the technical knowledge and skills required to set up one’s digital environments so as to reduce digital distraction are likely to be unevenly distributed (Hargittai 2002; Jenkin 2015). Hence, future research into individual differences in digital self-control should keep a wider socioeconomic perspective in mind, which could help identify study populations for whom research in this space may be particularly important (cf. Devito et al. 2019).
7.4 Revisiting what success looks like
We now return to the notion of ‘success’ for future digital self-control design patterns. Reflecting on the findings from existing studies and the present thesis, what might a desirable future look like?
The current state of research provides a rough picture of how a wide range of design patterns may support user self-control, with initial indicators of how main implementation details (e.g., how readily accessible and actionable usage visualisations are), and individual differences (e.g., distraction blocking may be more effective for users who struggle more with managing distractions at the outset) influence their effectiveness. While this initial work offers suggestive findings, an additional wave of high-powered, confirmatory studies is required to establish their reliability — preferably in collaboration with industry researchers (cf. Orben, Dienlin, and Przybylski 2019; Science and Technology Committee 2019) — before we can provide specific design guidelines that are rooted in robust research. What we can do at this point, however, is to use the initial body of evidence in conjunction with relevant theory and findings from general self-control research, to provide some guiding principles (cf. Shneiderman et al. 2018): in section 5.6.1, we used the dual systems framework and key findings from psychological research to generate clear predictions for the potential and limitations of two design patterns for self-control on Facebook. In the following, we extend this approach to provide a general guiding principle for design patterns aimed at assisting users in exerting self-control over digital device use.
7.4.1 From balancing screen time to empowering users to sculpt a balanced digital environment
As outlined in section 5.6.1, psychological research has shown that people who are better at self-control — perhaps somewhat paradoxically — use it less (Galla and Duckworth 2015). That is, people who are better at acting in accordance with their longer-term goals rely less on conscious in-the-moment self-control (System 2 control, cf. Chapter 3), and instead set up their environments to reduce exposure to distractions, and/or form habits that make their intended actions more reliant on automatic processes (System 1 control). As outlined in Chapter 3, a psychological explanation for this is that conscious self-control requires both (i) relevant longer-term goals to be present in working memory, and (ii) the expected value of control — which fluctuates over time with mood state and other influences — to be high enough to overcome the strength of competing impulses, which makes conscious self-control an unreliable strategy. It follows that, when designing to support digital self-control, it should be a main concern for us to empower users to adjust their digital environments such that the amount and motivational pull of the information they are exposed to remain within a range which — given their working memory limitations, reward sensitivities, and other relevant traits — allows them to effectively navigate those environments in accordance with their long-term goals, without being ‘overpowered’ by distractions.
Thus, in many situations, design patterns for managing time spent on devices overall and within specific functionality — which is what most existing studies have focused on — fail to address the root problem: to take the example of Facebook, a key struggle reported by our participants in Chapter 5 was inability to stay on task during use, largely because an excessive amount of engaging information on the newsfeed made them forget their original goal. This is not unique to Facebook; Lukoff et al. (2018) found in an experience sampling study that people tend to drift off-task during app use on smartphones, and that such drift is associated with finding use less meaningful. Thus, finding the right fit between the affordances provided by the user interface, and the user’s capabilities and goals, is key to digital self-control (cf. J. Cheng, Lo, and Leskovec 2017): when the cause of self-control failure is that the amount, and/or the motivational pull of, the information users are exposed to overpower their ability to stay on task, time management tools are unlikely to be the most appropriate design solution. Rather, a better solution is to provide tools for scaling down information amount and engagement level so that the user is able to effectively remain directed by their longer-term usage goals.
To be clear, time management interventions are appropriate in some circumstances (cf. Chapter 4‘s finding that ’time’ was the most frequent term in user reviews of DSCTs). For example, in the case of video games, if the user’s goal is solely to enjoy being immersed in the game, supporting self-control may simply amount to helping the user spend only his intended amount of time in that environment. However, in the case of Facebook, users’ goals are more likely to focus on accomplishing a specific task (e.g., messaging a friend, creating an event) rather than spending an allotted time on the site. In such cases, tools for time management (including those Facebook introduced in response to concerns over ‘problematic use’) are trying to solve the wrong problem, if what users struggle with is staying on task in the face of an interface that provides an endless stream of highly engaging content.
Focusing on users’ ability to sculpt their digital environment should naturally align with the goal of universal usability: as a profession, the goal of HCI is to address the needs of all users, across the diversity of human abilities, motivations, and personalities (Shneiderman et al. 2018). Similarly to usability principles of determining users’ skill levels, I suggest the following guiding principle for digital self-control design patterns:
Consider what level of information amount and attractiveness will be within a range that allows the user to exercise self-control and form their intended usage habits. Provide the user easily accessible means to adjust these factors to a level that suits them.
This level will vary between users, as well as for the same user over time – as particular habits for intended use are formed, the user may be able to handle a higher level of engaging information while remaining in control (cf. section 6.5.2).
Whereas design patterns such as usage visualisations, timers, and lockout mechanisms have dominated existing digital self-control studies (only one study, Lottridge et al. (2012), tested an intervention which made distractions less visually prominent), I therefore encourage future research to focus on design patterns which allow users to directly sculpt the amount and relative attractiveness of the information they are exposed to (cf. section 6.5.1). Such work may build on established usability principles of, e.g., using multi-layer approaches to handle multiple classes of users, with simpler ‘training-wheels’ interfaces being provided to novices (cf. Carroll and Carrithers 1984; Shneiderman et al. 2018). Specifically, such research efforts could study the effects of, e.g., by default hiding video recommendations on YouTube or Facebook’s newsfeed (cf. Chapter 5) and enable users to opt-in to video recommendations, or newsfeed posts, at varying amounts and refresh frequencies. This could lay the groundwork for interfaces that allow users to experiment with different amounts and engagement levels to find a ‘Goldilocks’ level where they can effectively reap the benefits of algorithmically tailored content without having their self-control ability overpowered. Such a line of work might benefit from engaging with the rich literature on attention management in aviation, which for decades have studied how to avoid distraction during navigation of complex information systems (e.g., Raby and Wickens 1994; Wickens and Alexander 2009; Wickens 2007, 2002), and has yet to be consulted in digital self-control research.
Ultimately, we may wish to work toward systems that are able to automatically detect the user’s goal and adapt the interface accordingly. For context, J. Cheng, Lo, and Leskovec (2017) found in a study of Pinterest that users reported roughly 50% of their use as being goal specific and 50% as goal non-specific, and that self-reported intent could be rapidly predicted from user behaviour. One exciting opportunity for future research therefore involves design patterns that would, for example, automatically make YouTube look different if the user goes to the site to find a cooking how-to video, compared to if the user visits the site to entertain themselves on the bus.
This approach readily connects with arguments to move from an ‘attention’ to an ‘intention economy’ (Searls 2012), in which people are able to shape their relationship with vendors on their own terms, and express their personal preferences, make choices, and participate in relationships in ways that are aligned with their intentions (cf. Harris 2016). Similarly, I would argue that devising effective means with which users can sculpt the affordances of their user interfaces, so as to match their unique needs and abilities in relation to self-regulation, should be a key consideration in future research on digital self-control design patterns.
7.4.2 Conditions for success - markets and regulations
In this thesis, we scoped our investigations to individual self-regulation. However, as noted in section 2.1.3, wider dynamics of the internet economy are main drivers of the way many current design patterns are effectively aim to undermine, rather than support, digital self-control. Therefore, when considering what it would take to move towards a future where users’ design needs are met, we need to look beyond individual users. Whereas any deeper investigation of the attention economy is not the aim of this thesis, I will in the following provide some brief remarks on what I perceive to be the main practical considerations:
Because we find ourselves in a world where a small number of tech companies hold disproportionate influence over how billions of users of digital technology direct their attention on a daily basis, it is highly important how these individual companies decide to resolve questions over digital self-control. As of yet, the ‘digital well-being’ tools introduced by Facebook, Google, and Apple all focus on visualising and limiting time spent on digital devices. Meanwhile, design solutions that would empower users to adjust their exposure to engaging information within services are only potentially available if services can be accessed in a browser and users are sufficiently tech-savvy to discover and install a suitable browser extension. In the case of YouTube and Facebook, this may not be surprising, as providing users easy means to remove or limit the output of recommender engines would not only relinquish control over expensively-engineered drivers of engagement, but do so at risk of revenue decline. In so far that providing such controls to end-users is important to digital self-control, some form of regulatory intervention may therefore be required to align companies’ incentives with users’ interests.
However, even though the UK government have tentatively explored regulation of ‘persuasive interfaces’ in relation to children’s use of technology (Wright and Sajid 2019), it is not clear what meaningful regulation might look like. Attempts to regulate design directly is challenging: regulation at the level of specific design features (prohibiting bottomless feeds?) is likely to be rapidly outdated by design innovations; and regulation at the level of more general principles (‘users shall be provided controls to scale down information amount and attractiveness’) may be too open to interpretation to be meaningful (cf. Turel 2019)
An alternative is to regulate in ways that incentivise market competition around desired parameters. For example, if users were in possession of their own personal data and companies competed over ways to interlink and interact with it (see Tim Berners-Lee’s vision for a re-decentralised internet (2019)), users might be able to choose between many different user interfaces to similar functionality, and select the product which best allows them to align their digital device use with their longer-term goals, given their cognitive abilities and personalities. This would contrast with the current situation in which users who wish to benefit from Facebook’s social connectivity are stuck with a user interface engineered to optimise revenue through extraction of attention and personal data. To change the status quo, regulators might mandate platform interoperability to enable companies to compete over creating user interfaces to similar data (cf. ‘adversarial interoperability,’ Doctorow (2019); in the US Congress, a recent bipartisan bill proposed to do just this, Mui (2019)). However, because opening up platforms also increases the risk that data are misused by third-parties, this road may contain obstacles, given recent privacy scandals associated with Facebook’s past practices of allowing third-parties extensive access to personal data.
Alternatively, regulators may consider digital design as analogous to, e.g., organic farming. That is, regulators may consider creating incentive schemes that make alternative business models that do not rely on extracting users’ attention and data more viable, and therefore make design goals aligned with digital self-control more likely. This might be achieved by, e.g., mandating that app stores prominently display badges disclosing companies’ business models, which could make it more appealing for consumers to pay for a product in which the developers’ design goals are aligned with their usage goals, as opposed to one which is optimised for extraction of their attention and personal data.
7.5 Next steps: Advancing digital self-control research through scalable action research
Much of this thesis invites follow-up research to, e.g., establish the robustness of findings and generate additional insights from explored methods. For example, as outlined in Chapter 4, additional insights might be extracted from the dataset of user reviews we collected from DSCTs in online stores, through additional thematic analyses of reviews sampled from tools implementing specific design features of interest. Similarly, Chapter 5’s study of interventions for self-control on Facebook was exploratory and should be followed by confirmatory studies, using effect sizes from the original study as a guide in power analyses for establishing a minimum sample size. In this section, however, we present a broader vision for advancing the state of digital self-control research, a vision that is centered around the Reducing Digital Distraction workshops (cf. Chapter 6).
Thus, just as it is instructive to be explicit about what we think digital self-control design patterns should achieve, it is useful to consider what research infrastructures would effectively advance research in this space. In this respect, two observations may be useful to keep in mind:
First, the challenge of supporting digital self-control is here to stay: as increasingly immersive digital technologies emerge — consider, for example, Facebook’s massive investments in VR technologies (Stein and Sherr 2019) — understanding how to design in ways that safeguard people’s ability to align device use with longer-term goals will only become more important. Therefore, our research efforts should be equipped to accumulate knowledge on user struggles and usage goals, as well as the effectiveness of design patterns for digital self-control, over time as digital technologies evolve.
Second, designing for digital self-control is an important and global challenge: as central activities — news consumption, entertainment, social communication — become digitally mediated, it is of real consequence that we discover effective design solutions and disseminate them effectively. Therefore, research efforts should consider practical impact, at scale.
However, with the exception of the Stanford HCI Group’s HabitLab, which appears to reflect an enduringly maintained infrastructure, all existing studies of design patterns have been one-off investigations. Moreover — again with the exception of HabitLab, as well a couple of studies that deployed evaluations in the form of university campaigns — most investigations have been small-scale and conducted solely for research purposes.
Chapter 6‘s study of the Reducing Digital Distraction workshops represent an initial step toward a research infrastructure which aims to (i) accumulate high-quality data relevant to central digital self-control research questions over the course of years, (ii) create positive impact by disseminating promising interventions to key audiences, and (iii) be able to scale. More specifically, this envisioned infrastructure consist of two complementary pieces: a workshop format, embedded within the student course offerings at the University of Oxford, and scaled via collaborations with other researchers and universities (cf. the recent push for ’many labs’ projects in psychology Klein et al. (2014)); and a complementary online format which builds on insights from the workshops and scales beyond student populations. Following the completion of this thesis, I will continue work with my collaborators on this infrastructure. Each element will be developed through a number of iterative steps:
ReDD workshops: refining, embedding, scaling
First, we will continue iteration on the workshop format and materials into a stable, thoroughly field-tested version. Afterwards, we will work with key stakeholders at the University of Oxford to include the workshops in the catalogue of welfare and study skills offerings available to students. Finally, we will collaborate with researchers and stakeholders at other institutions to extend the workshops beyond Oxford.
ReDD online: testing tasks, online workshop, scaling
First, we will conduct online studies of how key tasks from the workshops can be translated into an online format. Afterwards, we will develop an online-only version of the workshops, which walks the user through a process of reflection and intervention selection. Finally, we will widen the focus of the online offering beyond students, and incorporate intervention recommendations based on accumulating evidence from the workshops and online studies.
In other words, the initial key step is to embed the ReDD workshops within the infrastructure of an educational institution. This may not only allow the workshops to reach a larger number of students, but also enable research data to be collected long-term within an institutional structure that is more resilient to the ebb and flow of research funding. Thus, by developing a format which helps address a student need, we hope to piggyback on existing infrastructure, establishing an enduring research tool, that may subsequently be exported to other institutions and beyond. Eventually, we will scale this effort to broader audiences via an online platform which distils evolving findings into a high quality tool for exploration and recommendation of targeted interventions, and for advancing digital self-control research.